To the consumer, the weather forecast can often seem about as reliable as a fortune teller. Instead of rain, we end up with sunshine; we’re expecting blue skies, but hailstones fall on our heads. How can we rely on these forecasts if exactly the opposite can occur? Working with weather and climate forecasts becomes even more difficult when significant decisions depend on them, such as if a company is trying to find the best site for a wind farm. Will it still deliver electricity in 30 years?
Ludwig, 29, leads the independent research group “Machine Learning in Sustainable Energy Systems” within the Cluster of Excellence “Machine Learning: New Perspectives for Science” at the University of Tübingen. Together with her team, she develops models that use machine learning to help predict, for example, how much wind and solar energy can be gathered at a particular location. Her office contains nothing except a whiteboard covered with mathematical equations. As she explains it: “we need to have an energy network where input and output are balanced. To achieve this, we need to know both sides well: the demand side (i.e., the consumers), as well as the supply side, for example how wind turbines react to changes in the weather.”
Computations as basis for political decisions
These days, Ludwig is focused on the supply of electricity. She takes up her tablet and paints a grid pattern of thick red lines with her finger. “Imagine that this grid covers the whole world,” she says. “In the climate model, we have data for each grid point, each intersection. But for the white areas between the lines, we can only guess.” Wind speed, for example, is different in a forest, a city or a field. And the forest will change too, depending on how the climate develops in the future. Between 20-100 variables usually contribute to the algorithms’ calculations, including fixed variables such as the turbine’s height and the size of the rotor blades, as well as varying elements such as weather and climate.
At the moment, Ludwig and her team are trying to determine which models and which variables predict the future most accurately. According to Ludwig: “We would like to keep the range of possible outcomes as small as possible.” That would allow the calculations to be used as the basis for political decisions. In Germany, where the placement of every wind turbine is a subject of dispute, it is important to put the most effort into those locations and projects where the conditions actually make it worthwhile.
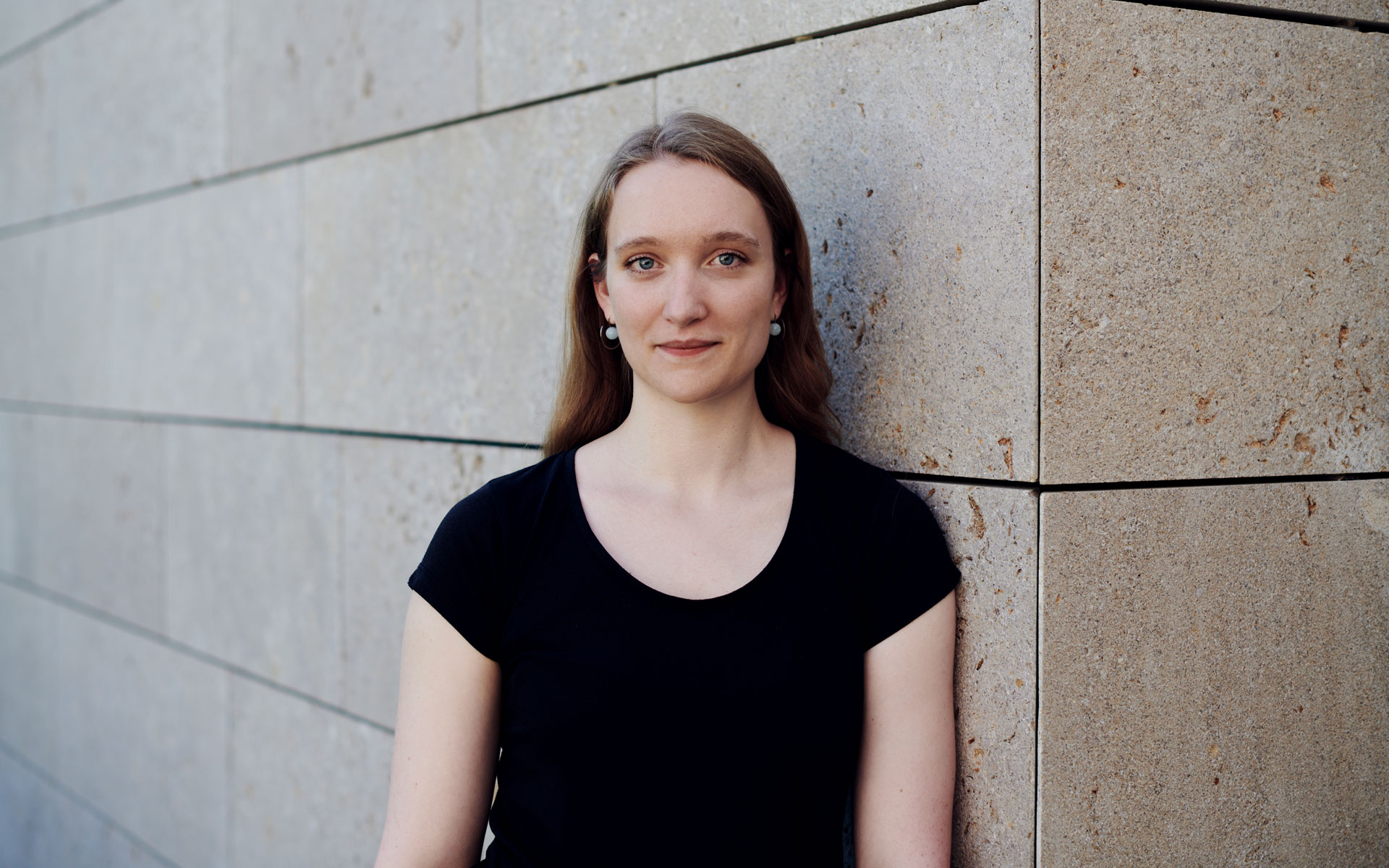
Ludwig develops models based on climate data that help predict the future energy supply. © ELIA SCHMID / UNIVERSITY OF TÜBINGEN
When Ludwig applied for the job at the Cluster of Excellence two years ago, she brought the topic of renewable energy systems with her. She has always been interested in topics that are relevant for society and politics. At university, she was fascinated by the idea of understanding society with the help of statistics. “It felt like meaningful work,” she says. Because you can only work out and resolve problems that you know well.
Ironically, she never liked maths in school and preferred languages. Today she says: “It has taken a long time for me to understand that you can be good at both languages and science.” In the end she studied economics at university, did her master’s in information systems and network economics, and her PhD in computer science at Karlsruhe Institute for Technology. Today, she says: “I’m still lousy at doing maths in my head, but when it comes to proper maths, I’m good.”
Ludwig got interested in energy topics during her bachelor’s thesis when she researched energy prices. Later, she worked on renewable energy systems with a focus on energy consumption for her PhD dissertation. She proposed algorithms that would help consumers use electricity at the same time as it is being generated by a renewable energy source, for example using the washing machine while the sun is shining. In Germany, such a change in energy usage by a single household would not have a noticeable effect on general consumption, but it would be significant if a large manufacturing company made the change. As an example, Ludwig applied machine learning models to calculate how a factory for electronic components could operate more efficiently. She found that postponing just two or three processes by 10-30 minutes could reduce peak consumption by a fifth. “It was remarkable to see how much can be achieved through even small changes at the right point,” she says. Until very recently, the problem was that energy costs were generally too small a factor in most companies’ overall operating budgets. “We have to wait and see what effect the current energy crisis and the price increases will have.” Will companies take the initiative and adapt production to renewables? Ludwig is sceptical. “I think the government has to offer incentives and impose standards.”
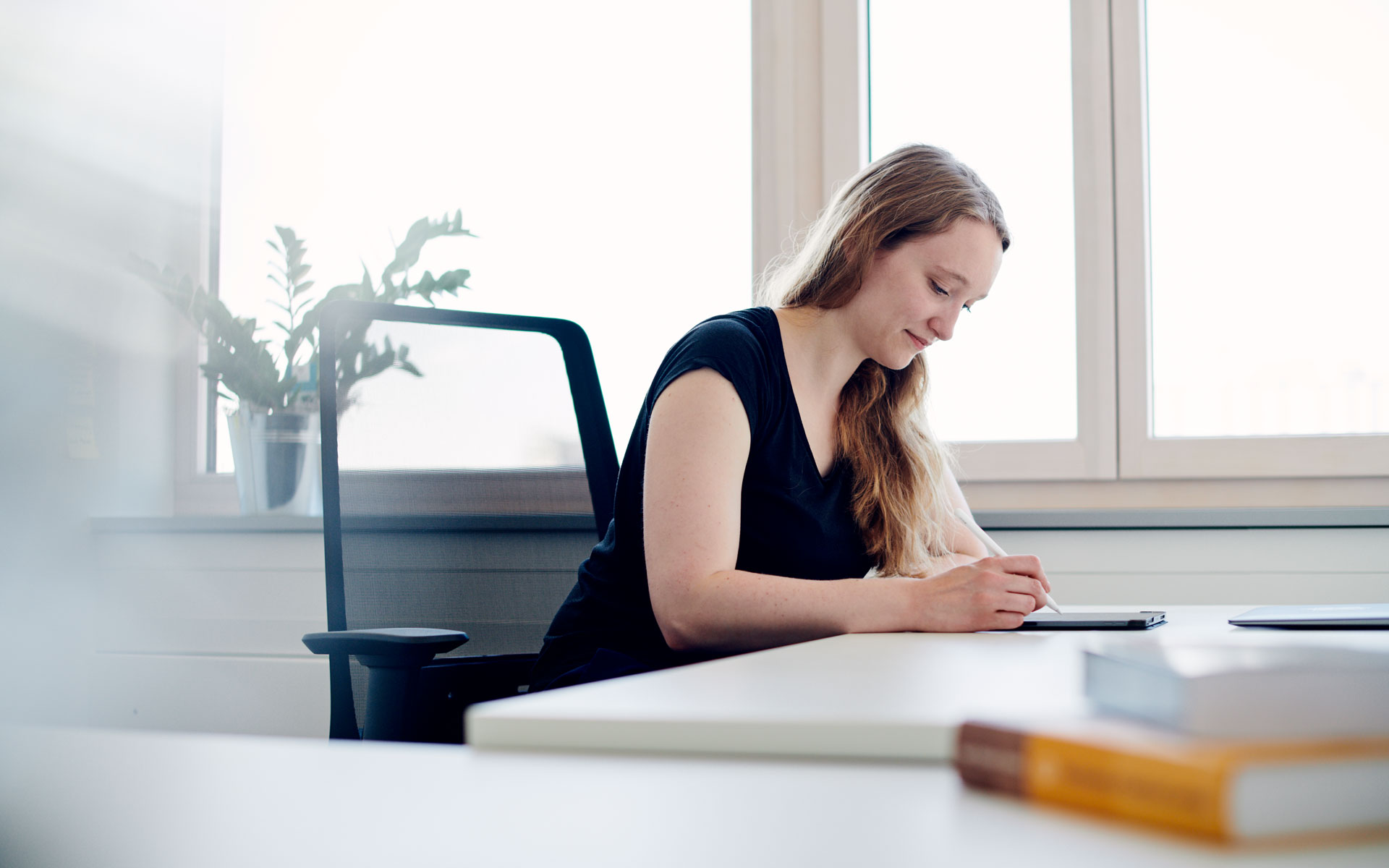
Models will always contain some uncertainty, but they can be the basis for political decisions. © ELIA SCHMID / UNIVERSITÄT TÜBINGEN
An Algorithm is only as good as the data
At present, Ludwig is working on the interplay of weather, climate, and the energy system as well as on the uncertainties of predictive models. In her field, an algorithm is trained on historical data. For example, it might look at energy and weather data from 2019 and 2020 and determine the links within the data. Then it would make a calculation to forecast how much electricity will be produced under what conditions in the future, (e.g., 2021, but maybe also as far ahead as 2050). Ludwig then analyses the outcomes. How well does the algorithm predict future developments? Of course, an algorithm is only as good as the data it has learned from. In the case of predicting energy supply and demand, it depends on, among other things, climate and weather models. The problem is that weather models often overestimate how certain their predictions are. But Ludwig has demonstrated that if we factor in this overconfidence, the models’ predictions of future energy production are not only more precise but are also better able to estimate their own levels of uncertainty. The researchers can then better assess the likelihood of the algorithm’s predictions actually coming to pass.
Models will always contain levels of uncertainty
In general, when studying machine learning an important objective is to state as clearly as possible how uncertain a model’s prediction is. But doing so is very tricky, because the further the algorithm-based models predict into the future, the more uncertain their predictions become. Nobody, for example, knows exactly how the weather will change, when tipping points will be reached, when the permafrost will have thawed, or when the arctic ice shelves will have melted. There could be more storms, and then the wind turbines might have to be switched off more frequently.
Models for future energy supply will always contain some level of uncertainty. “Politicians and entrepreneurs are often reluctant to base their decisions on algorithm-based models,” Ludwig says. They want clear, unambiguous statements – and that is difficult for scientists. In the coming months, Ludwig and her team plan to discuss their work more publicly and to communicate the uncertainties involved. She is planning to try out new, creative methods as well, such as a collaborative project with design students. She wants people from all walks of life to understand the huge potential machine learning has for the supply of renewable energy. She is convinced that there is generally some public interest in her research. “People have realised that an energy system based on renewable energy is possible. We shouldn’t wait until the global temperature increase has already reached the limit of 1.5 degrees.”
Translation into English: Fortuna Communication
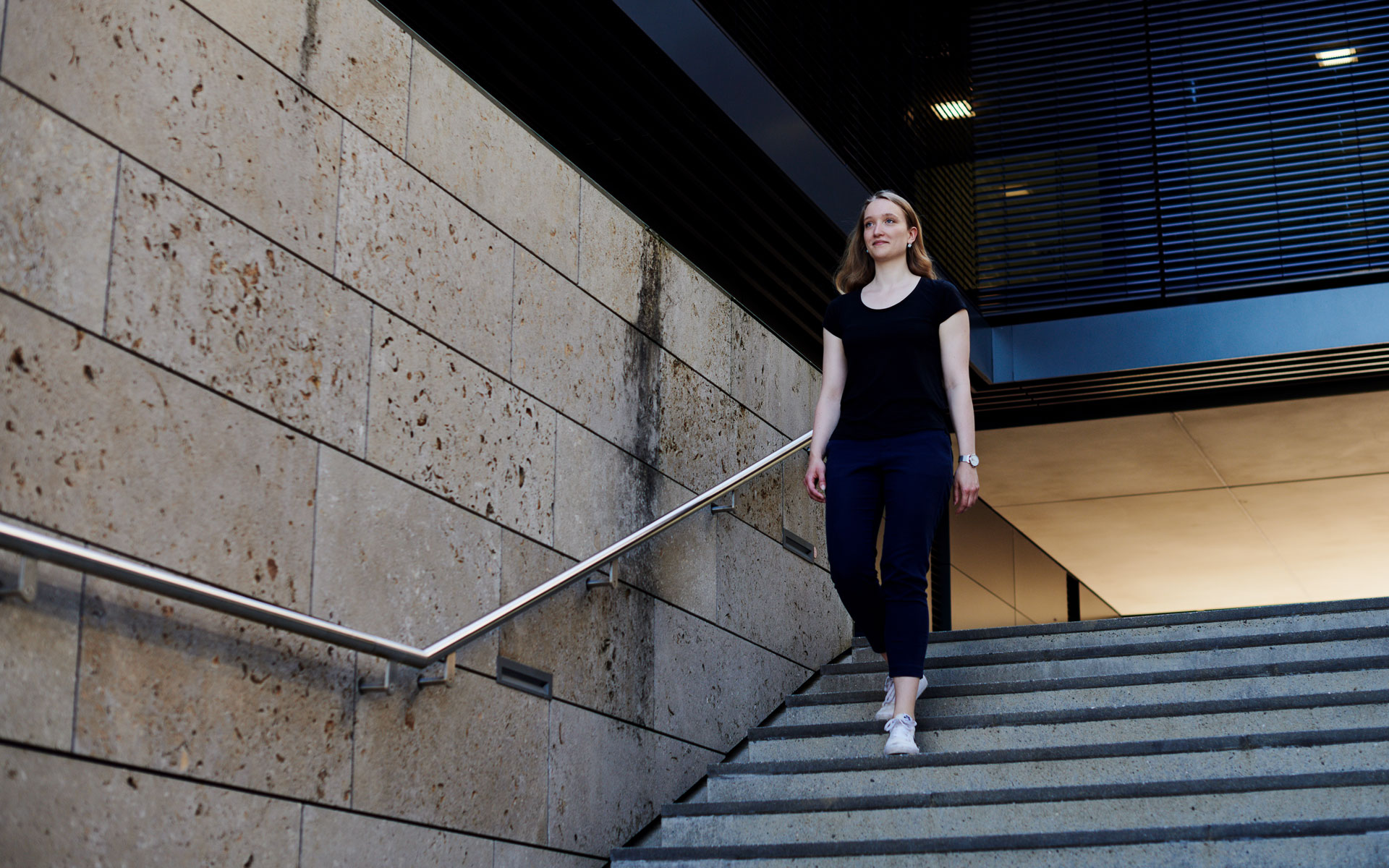
Ludwig wants to raise more public interest for her research. Therefore she is currently working on an art project with design students. © ELIA SCHMID / UNIVERSITY OF TÜBINGEN
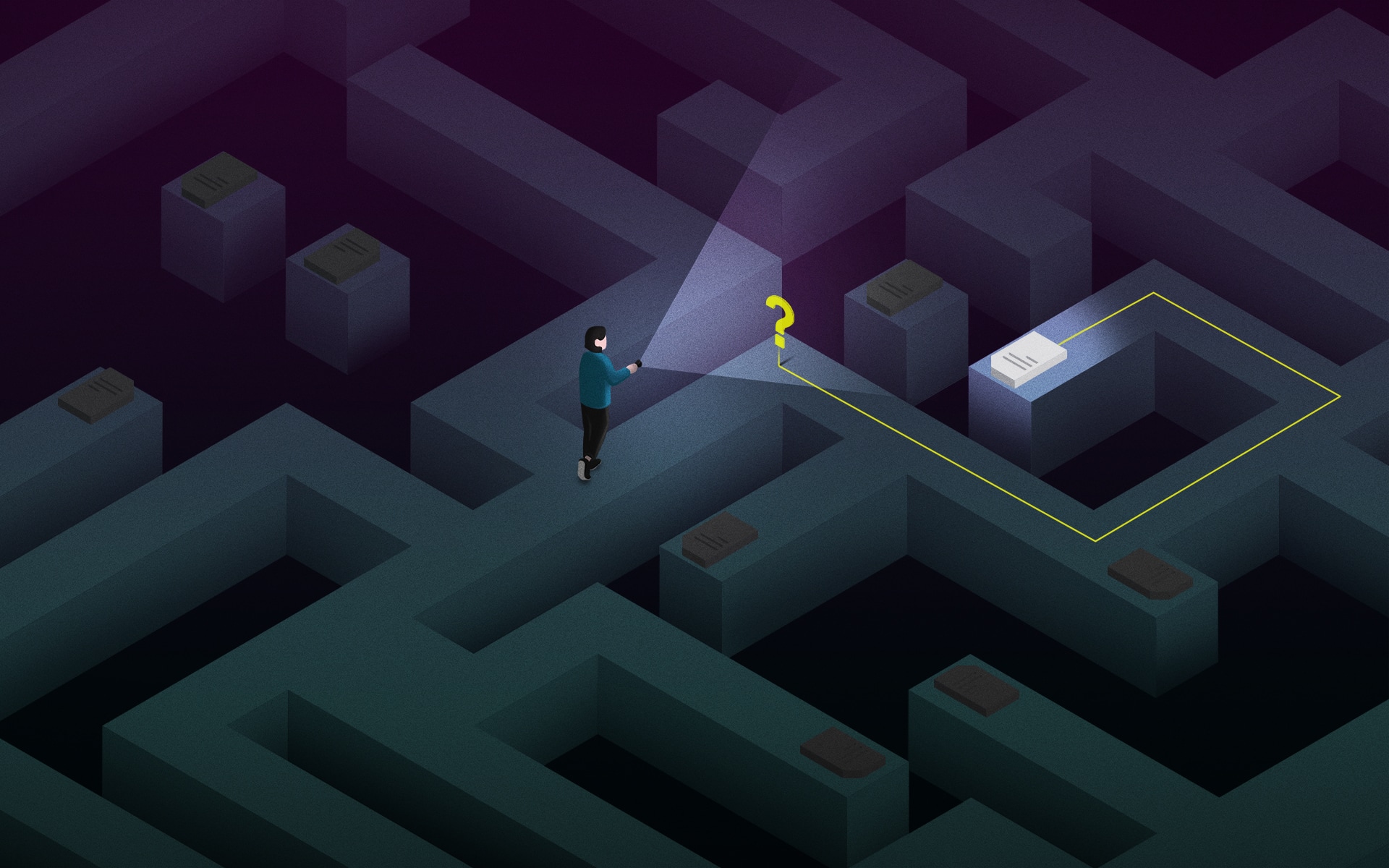
Artificial Intelligence (AI) – Should it explain itself?
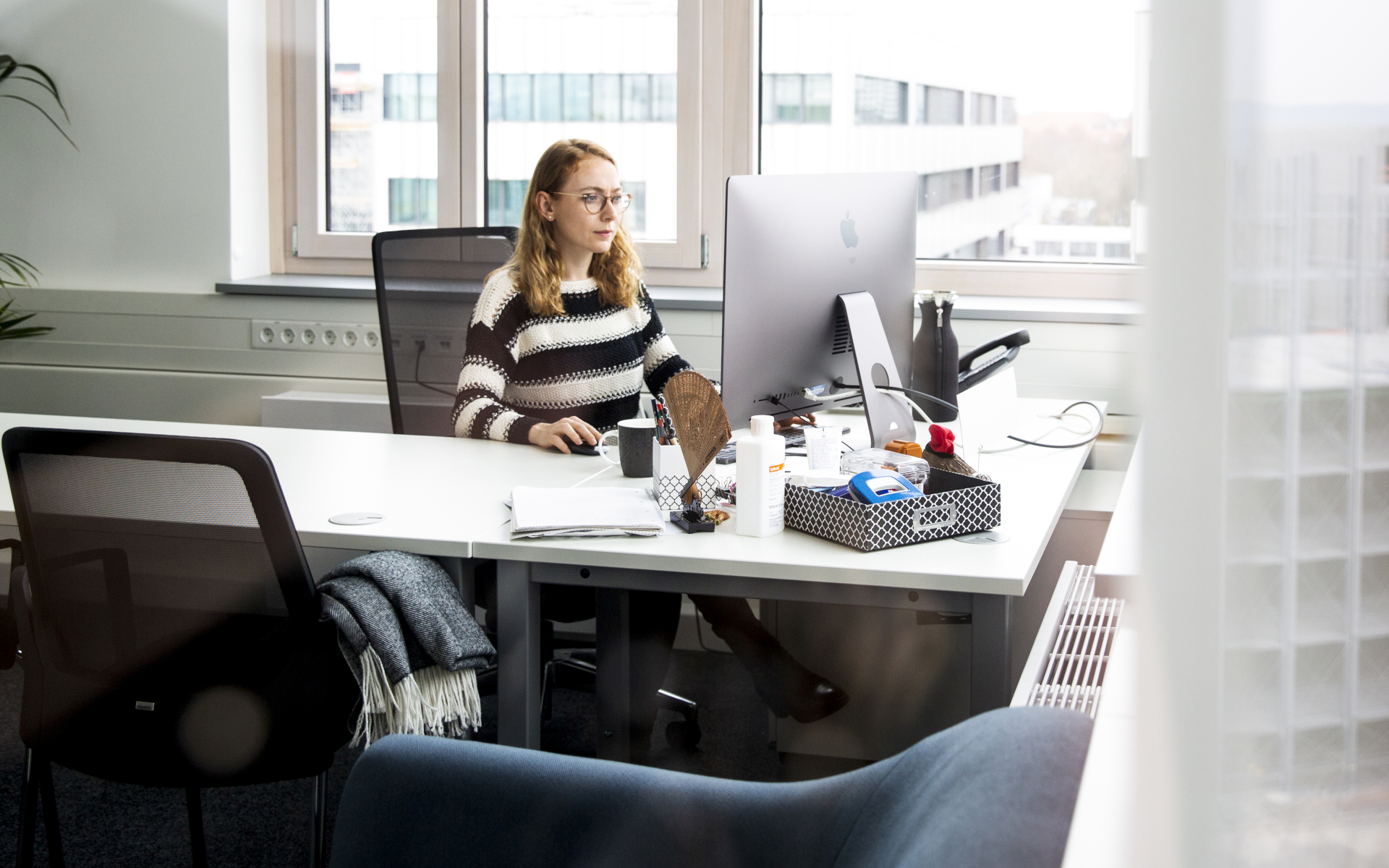
Towards AI systems that can explain decisions
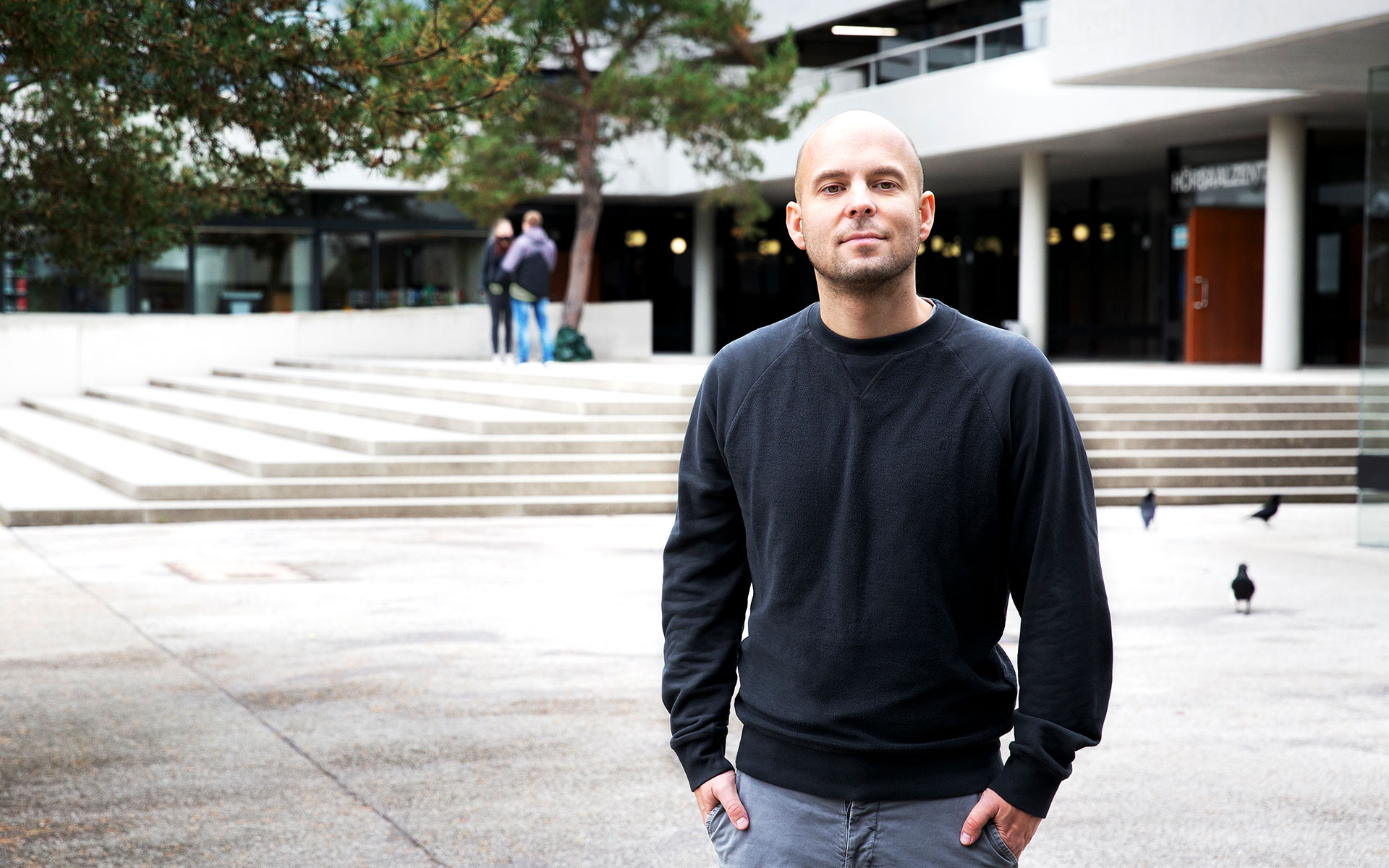
Comments