When it comes to illustrating the benefits of machine learning (ML) for research and society, medicine certainly can be considered an ideal showcase discipline at the moment. Thanks to the breakthroughs in automated image recognition, there may in the meantime be an algorithm for nearly every illness with externally observable symptoms. These algorithms then allow medical specialists to diagnose with precision. Equally impressive are various models for predicting health risks. With their help – in cases such as circulatory failure or kidney failure in intensive care patients – timely intervention becomes possible that could save many lives.
Does the algorithm really make the better diagnosis?
Starting in 2016, a few high-profile studies laid the foundation for the hype surrounding ML in medicine. In these studies, ML algorithms were to determine illnesses by means of clinical images. As a benchmark, the accuracy of the algorithm was compared with that of specialists. The tenor of all the studies was basically the same. The algorithm was at least as good as or even better than its human counterpart. Reports in the media then fired up an image of rivalry between humans and machines. At times, you could get the impression that people would soon have to make way for the algorithms surpassing them. The engineering feat behind the training of algorithms is certainly remarkable. But even when the presumed advantages of the algorithms are apparent in clinical practice, the studies nevertheless do not warrant the assumption that the algorithms in question actually bring benefits.
That’s also due to how the studies were designed. First of all, they were tailored to the strengths of the algorithms. The human specialists had to base their diagnoses solely on clinical images. Other modalities that are fixed elements of clinical practice (patient records, medical devices, direct information from the patients, etc.) were not available to them.
What’s even more significant is that these studies set an antagonistic framework between specialists and algorithms. Conclusions about the interplay between doctors and algorithms couldn’t really be gained from those results.
Yet in the scientific community there is a consensus that the task of algorithms should not be replacing, but supporting doctors – for both technical and ethical reasons. That’s why it’s time to shed the antagonistic understanding of the relationship between doctors and algorithms. In the interest of furthering medical advances, we should instead be focusing far more on the interplay between the two. That’s because the success of this interaction is the precondition for ML coming into daily clinical use to a certain degree at all.
What problems occur during interaction between algorithms and doctors?
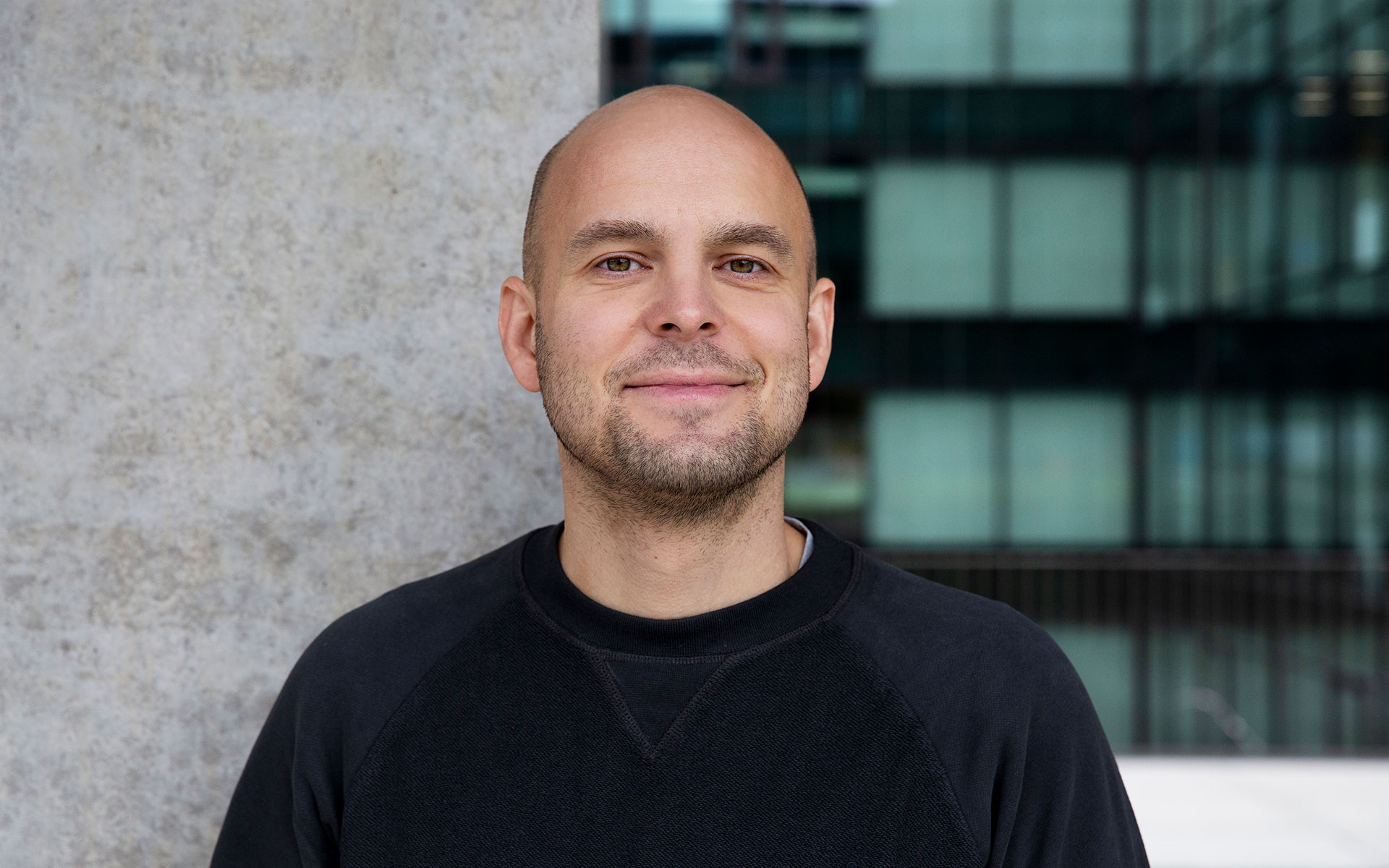
Thomas Grote, a philosopher specialized in technology © SOPHIA CARRARA/UNIVERSITY OF TÜBINGEN
The main challenge of interaction between doctors and algorithms is that two agents are involved. Each reasons in different ways and therefore has divergent limitations. Describing algorithms as agents is related however to its purely functional role in this case, meaning that it’s not a matter of ascribing it higher-level intellectual capabilities.
To simplify this roughly, ML algorithms make conclusions by developing mathematical decision-making rules. They use these to link input (a set of known variables) and output (the prediction/classification of a certain disease). Doctors use a combination of implicit knowledge, varied heuristics, and statistical procedures to make their diagnoses. Therefore, algorithms and doctors are prone to make different mistakes respectively. The robustness of ML algorithms suffers as soon as they are confronted with data that deviate from the training data. Doctors, by contrast, demonstrate deficits when it comes to calculating risks.
In addition to the different style of reasoning, there is a problem in the interplay because doctors make themselves excessively dependent on algorithms. A series of studies has shown that novices in particular tend to take on the diagnosis of the algorithm, even when their own diagnosis diverges from it. Especially when the algorithm’s diagnosis is wrong, there’s a risk doctors will be misled, in that they subordinate their own diagnosis to that of the algorithm. A consequence is that algorithms may undermine the (epistemic) authority of doctors. That then creates other problems. Particularly in situations where uncertainty is present with respect to the diagnosis, doctors could be tempted to decide defensively. Especially with novice doctors, this may make the step towards becoming an expert more difficult.
How must the algorithm be designed to ensure a successful interaction?
The challenge for ML research is consequentially to create ML algorithms that optimize interaction with doctors. To do this, it’s necessary to understand the mechanisms that allow doctors to make better decisions based on algorithmic diagnoses. This can be illustrated using explainability of ML algorithms as an example.
Reasonable explanations work as a connecting link between doctors and algorithms. The function of the respective explanations is that they form a basis for confidence in algorithmic decisions. Central to this is that the algorithm’s incorrect diagnoses are discoverable. This is a difficult undertaking if you consider the time pressure doctors frequently are under when making decisions as well as the often insufficient medical knowledge with respect to the etiology of diseases. It follows that the success of the explanations can be assessed by seeing if doctors interacting with ML algorithms make better diagnostic decisions with them, than both agents do working independently.
Explanations play a decisive role for successful interaction between doctors and algorithms in that they justify the diagnostic decisions of the algorithm. In doing so, the explanations fulfill different functions. One is developing confidence. Explanations will allow doctors to determine if the diagnosis was made in a reliable manner. Another is that explanations make the algorithm assailable, because possible reasoning errors become identifiable. Beyond that, for novice doctors, the explanations also have an educational function: through them the doctors learn to direct their attention to the relevant characteristics of medical images used to diagnose disease.
Nonetheless, it has to be noted that – to my knowledge – there are as yet only a few studies that have systematically examined the effect of algorithmic explanations in medical diagnostics.
What is philosophy’s significance?
The development of algorithmic explanation models in a medical context is in any case particularly complex. Meanwhile, in ML research, there are a number of approaches that already exist to make opaque ML models explicable – whether it’s the visualization of conspicuous features or by ranking the most important statistical characteristics leading to a diagnosis. A key question during this process is how and what exactly is being explained with these approaches – and what isn’t! Take for example, using a heatmap to illustrate which characteristics an algorithm focused on while analyzing an image of a retina. What information can be derived from that? At the same time, to what degree can such visualizations mislead or promote confirmation errors? This is the point where the technical side of ML research hits its limits. Precisely epistemology (the philosophical discipline dedicated to concepts such as knowledge, understanding, or justification) and the philosophy of science have a rich well-spring of experience in critiquing the explanatory capabilities of models. Epistemology can apply this to develop important stimuli for the development of epistemic criteria and carry out evaluation of current methods of algorithmic explanations. I see another possible contribution of philosophy, more concretely, in that it will specify what possible functions algorithmic explanations should fulfill and what the conditions for success of the respective explanations are. What is more, philosophy will evaluate the degree to which existing technical solutions actually meet these demands.
To ensure that philosophy can make a rational contribution, it’s necessary to address medical research very precisely (What is actually the logic of diagnostic decision making?) and ML (Which explanatory approaches are actually possible in principle?). In short, improving the interaction between doctors and algorithms requires a multi-disciplinary approach.
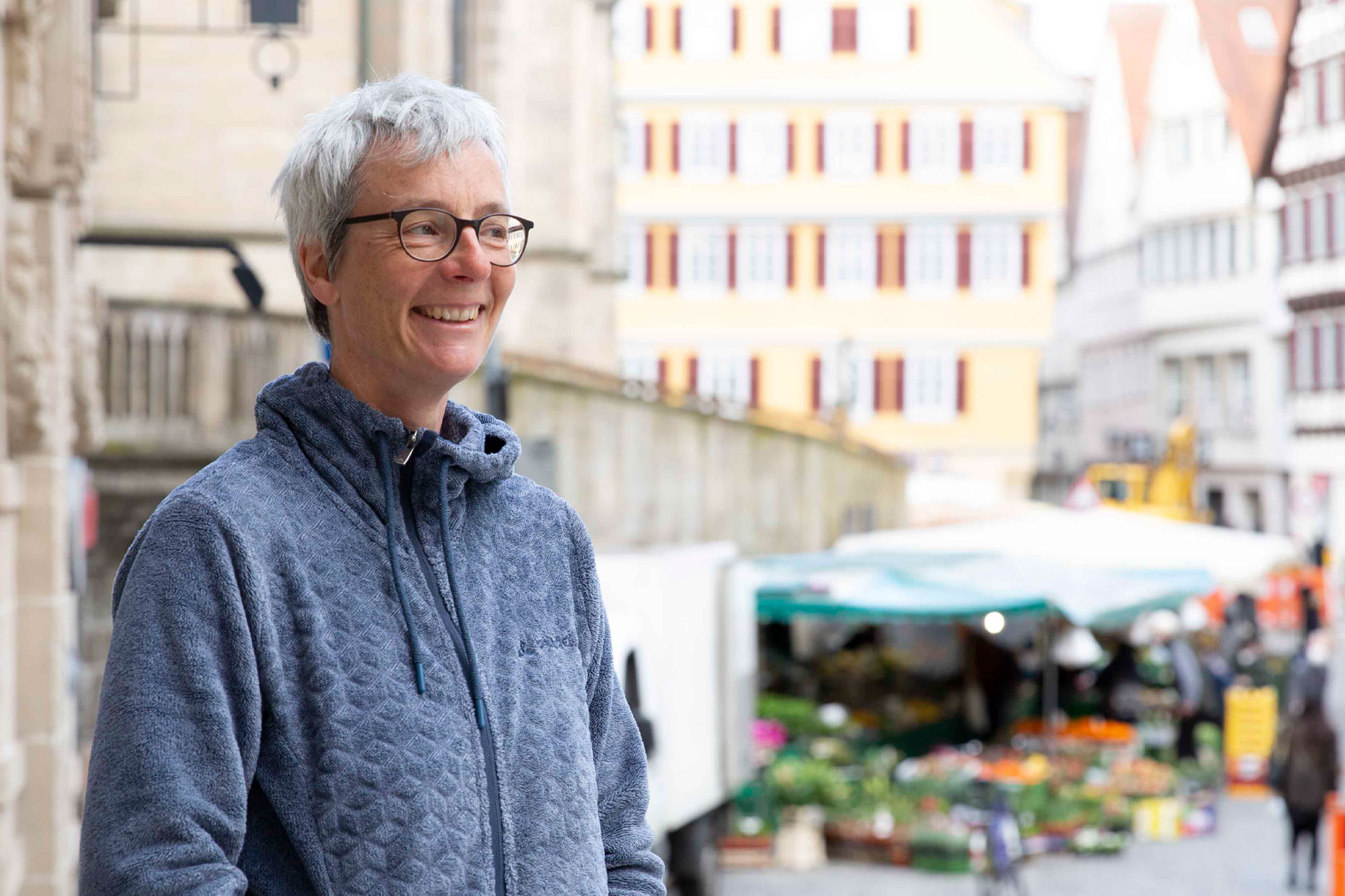
Responsibility Cannot Be Delegated to an Algorithm
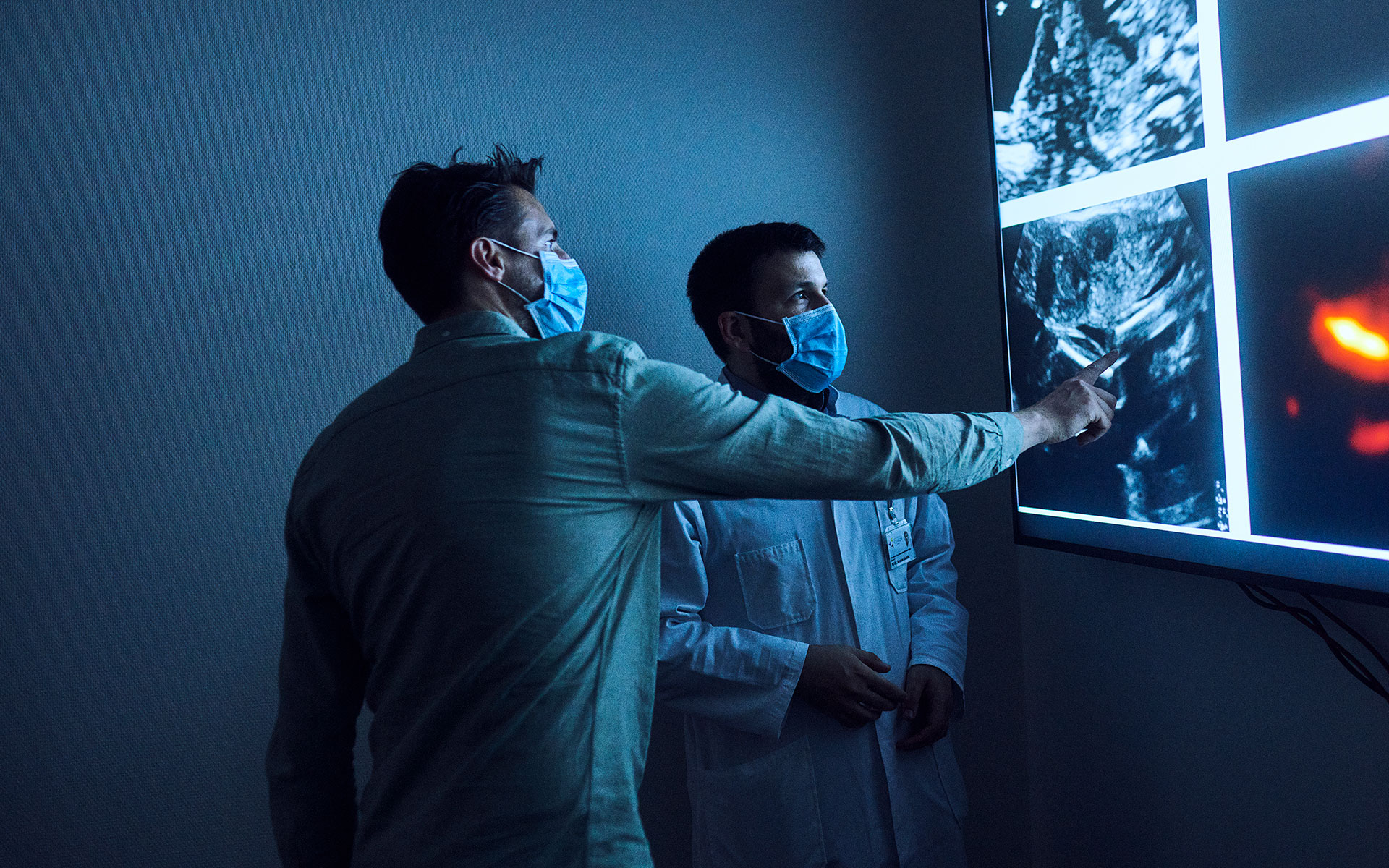
When Artificial Intelligence Predicts a Heart Attack
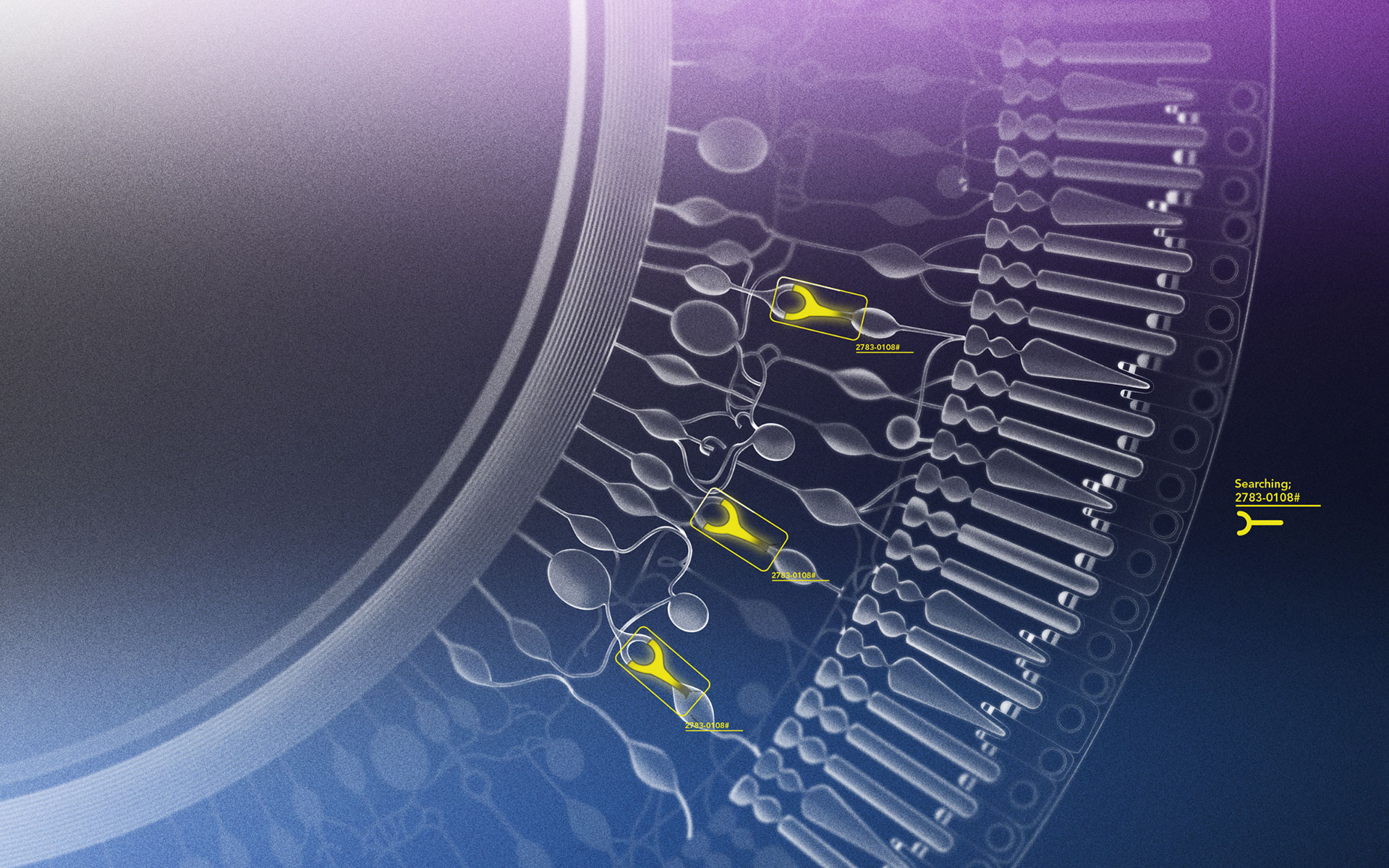
Comments