Many AI systems are seen as “black boxes.” They are murky systems – decision-making mechanisms that lack transparency. What would it be like if we could interact with the system, almost have a little chat with it? What if we could question the results and have the system explain its choices?
Zeynep Akata’s research has already spotlighted precisely this seemingly fantastic experiment in thought play for several years now. The 35-year-old Professor for Explainable Machine Learning at the University of Tübingen says, “Thinking about explainability from the user’s perspective is really necessary.” Akata continues, “people can form connections with machines.” Her research examines the various facets of explainability – namely, the ability of a learning machine to justify its decisions. Ultimately, this is to provide insight into AI’s decision-making processes in order to strengthen user-confidence in the machine.
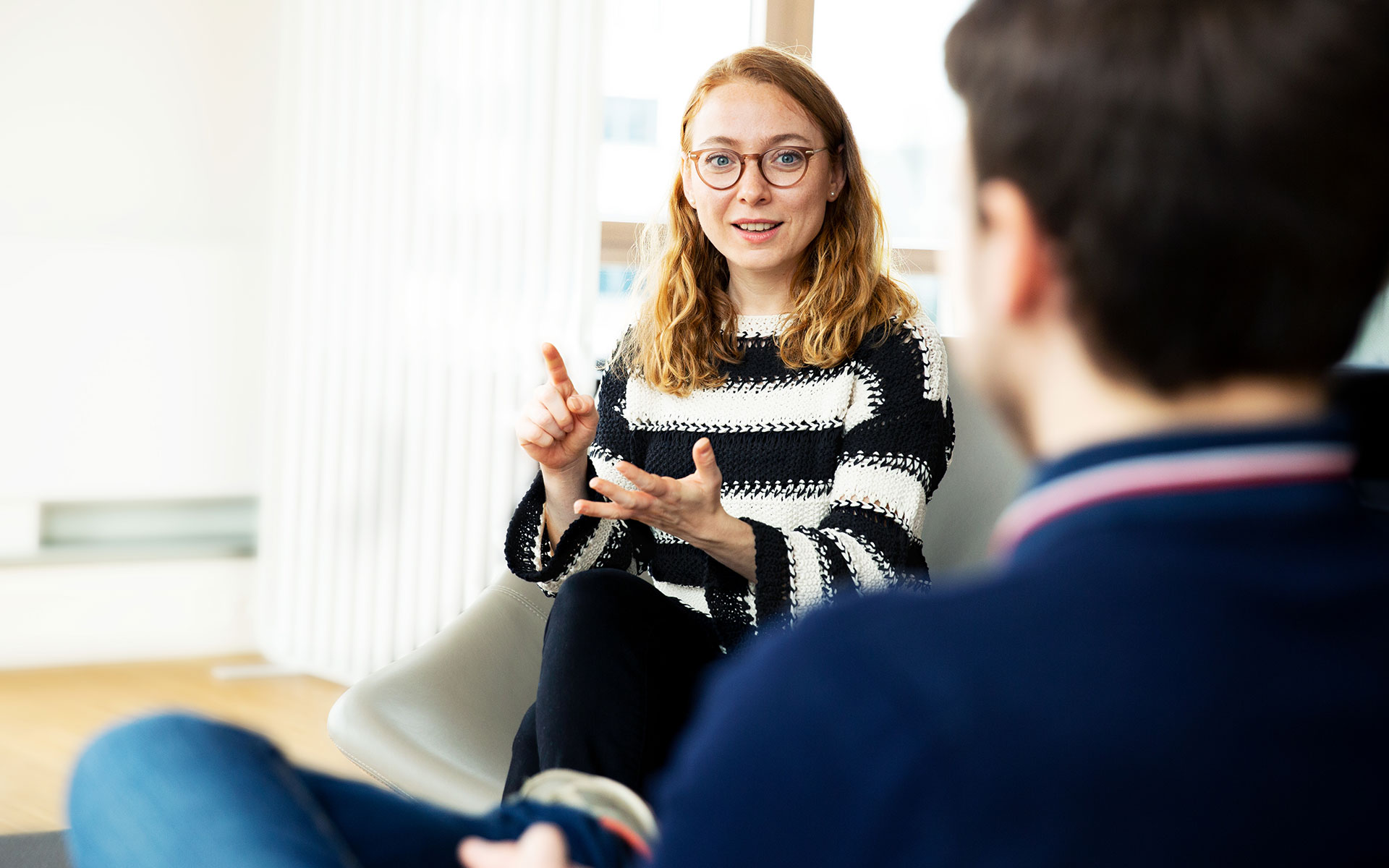
Akata aims to give insights into the decision-making processes of AI systems. © SOPHIA CARRARA/UNIVERSITY OF TÜBINGEN
Explainability has gained in importance extending far beyond scientific research in recent years. Politicians have discovered the issue and made it part of their agenda. For instance, the 2018 EU General Data Protection Regulation (GDPR) is interpreted as introducing “a right to explanations” regarding automated decisions. When an automated decision has been made, the affected person must be able to obtain meaningful information about the logic on which the system is based. In a white paper published just this spring, the European Union called for AI systems to be more transparent and reveal the pathways by which decisions were reached.
Attention maps show image area that is system focus
So, how exactly, do you make a system more explainable? Zeynep Akata illustrates this by referring to AI systems that recognize and classify images. Based on deep learning, these systems use artificial deep neural networks modeled on those of the human brain and are just as difficult to decipher.
“In principle, there are two types of explainability,” Akata says. “One is looking inside the system, called introspection, and the other is justification explanation.” Imagine looking inside the system as opening a person’s head to see which neurons are activated during a particular thought process. This isn’t all that easy in reality – neither with humans nor machines. That’s why researchers are looking for other ways to understand how the machine proceeds, for example, by means of an attention map. On the map, the system uses colors to chart the amount of attention devoted to specific parts of the image. Red signifies the most influential region, for example, followed by orange, then yellow, and so on. This way, humans can see the parts of the image the machine has focused on and to what extent, allowing people to largely comprehend how the machine proceeded. It also allows them to recognize errors in the machine’s processing and understand why it is delivering incorrect results.
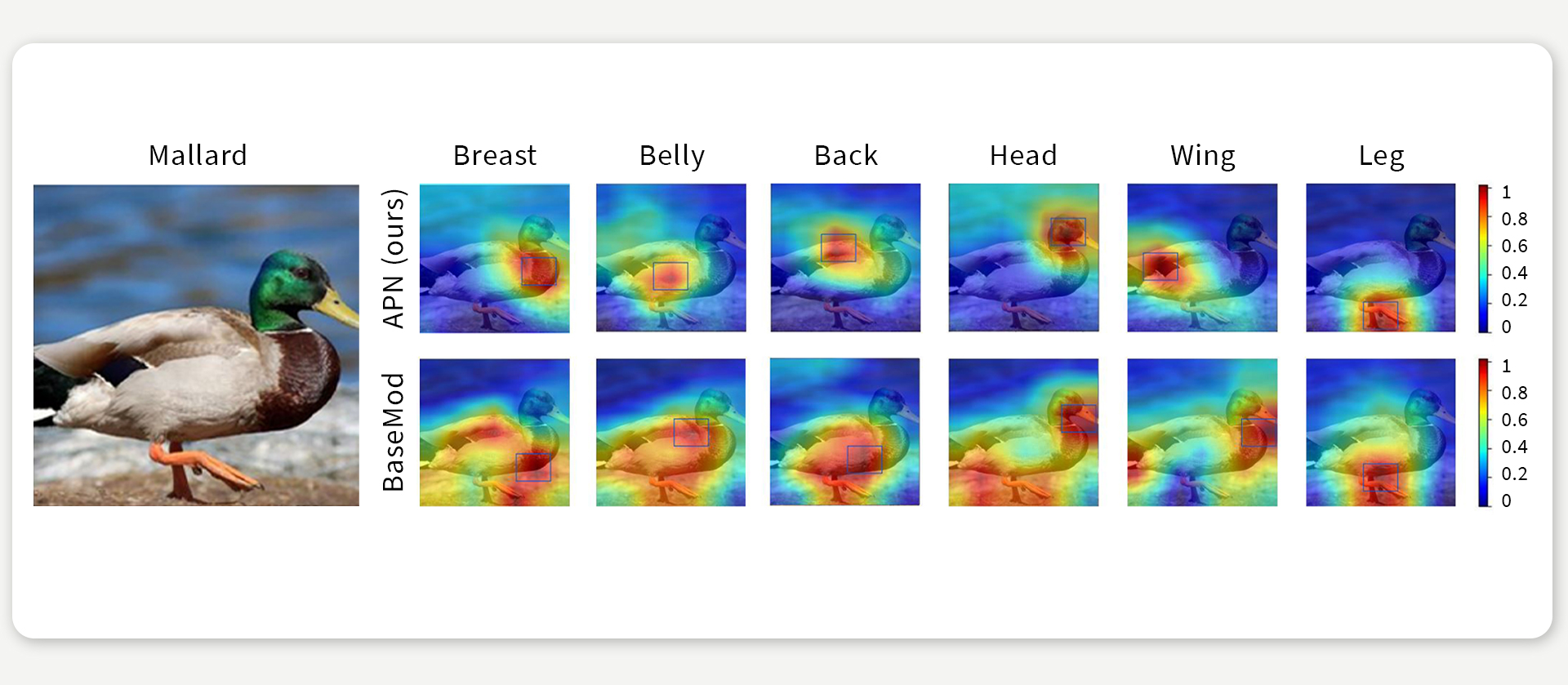
Attention maps allow to look inside the system. In this example, two algorithms should determine different body parts in the image of a mallard. The attention maps illustrate that one algorithm (APN, developed by Akata and colleagues, top row of images) accurately detected the crucial image region in all cases and accordingly predicted the body parts more precisely than the other algorithm (BaseMod, bottom row of images). Figure according to Xu et al, 2020.
Akata explains the second category, justification explanation, using the example of a system that allows users to ask questions about an image, such as, “What type of bird is this?” The system should name the class-specific features – such as a red body, black face, and red beak – and label them within the image by placing a box around them. Akata’s work is special because the system can even provide its answers in natural language. It replies to the question about the bird’s species with: “It is a cardinal because it is a red bird with a red beak and a black face.”
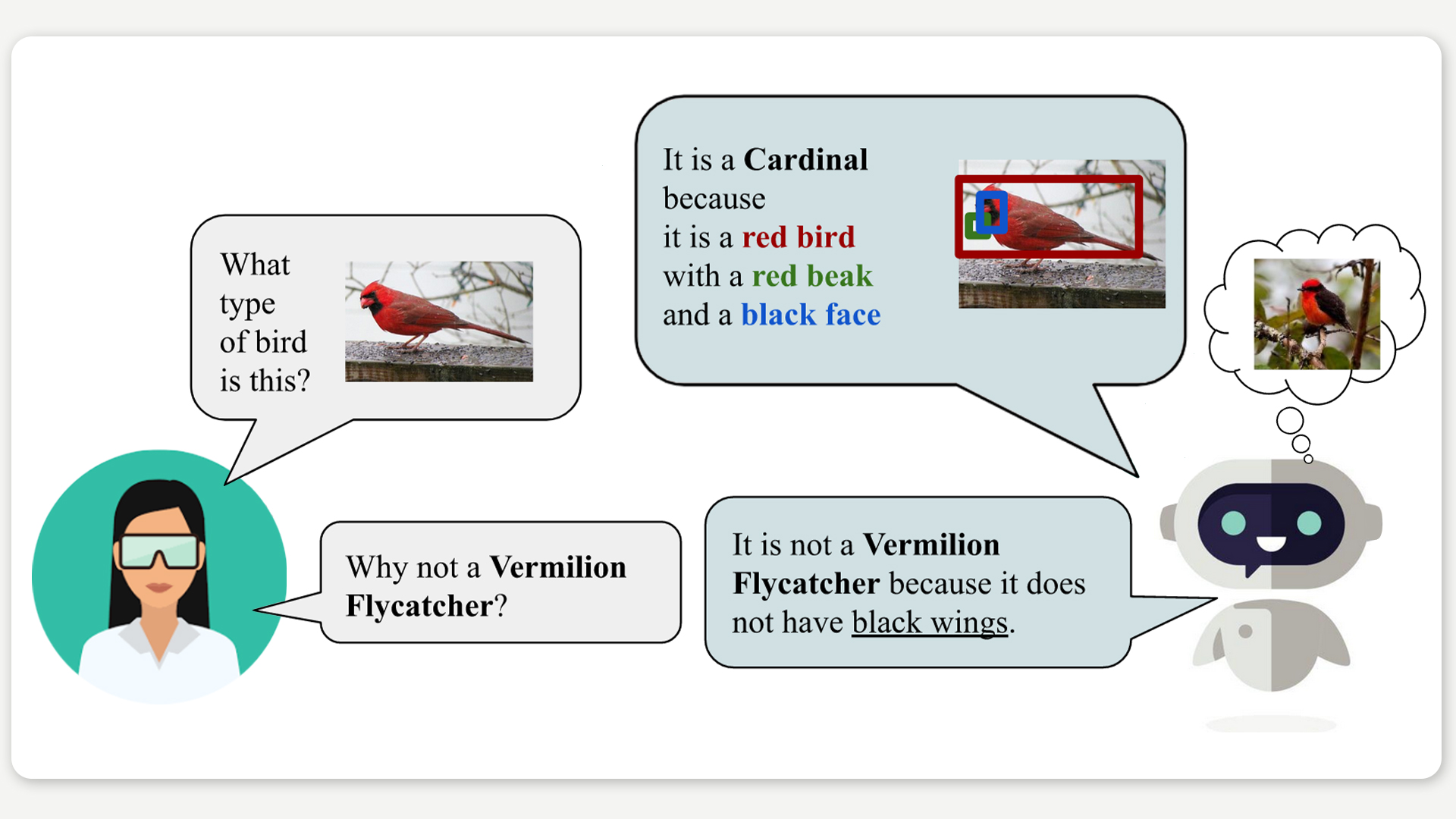
Akata is conducting research on AI systems that can explain their decisions in natural language. Even a small conversation between user and system thus becomes possible. Figure according to Hendricks et al., 2018.
Explainability can bring humans and machines closer together
An advancement like developing a system from a black box to procedure that can be questioned, has the potential to increase decisively people’s trust in AI systems. “That’s the closest we can get to human communication,” Akata emphasizes. “The system must provide coherent statements and point to the correct attributes in the picture. It’s like questioning a witness in court to determine whether he or she is consistent or not,” she explains.
This comparison elucidates what Akata’s research is about – her aim is to disclose technical processes and give technology users new agency in the decision-making process.
Akata has found the connection between explainability and natural language fascinating since it emerged as a research topic. This dates back to around 2015, when she was a postdoc at the Max Planck Institute for Informatics in Saarbrücken. She was researching computer vision with Bernt Schiele and his team, working on systems that can predict – that is, recognize and determine – features in images. “We were using these features as a means for improving our classification models, but they can be used as a means for explanation, too. So, I wanted to collect data and take it a step further and generate natural language explanations,” says Akata. The pioneer in this field, Trevor Darrell, was a professor at the University of California at Berkeley. Akata did not know him personally, but she approached him when she saw him by chance during a break at a major conference. He was standing there reading something on his cell phone. “I said to him, ‘I have a data set and an idea, I need your help.’ He lifted his head and looked at me as if he wanted to say, ‘Who are you?’” Akata laughs as she tells the story, as if she can still see the man’s astonished look right before her eyes. But her determination simply to approach Darrell spontaneously paid off.
Chance encounter charts new paths
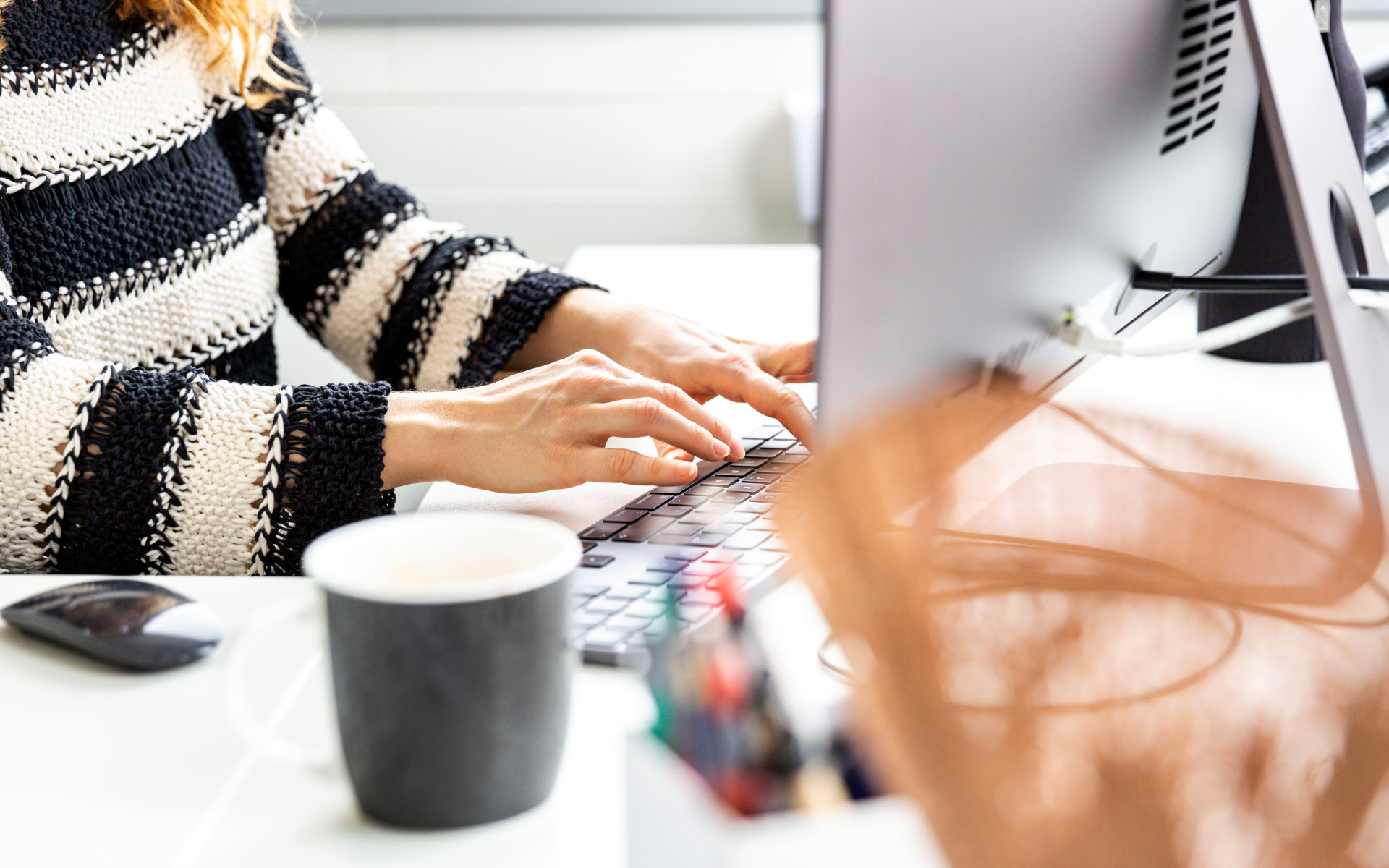
Explainability has the potential to increase trust in AI systems. © SOPHIA CARRARA/UNIVERSITY OF TÜBINGEN
Looking back, that encounter marked the start of an exciting time – and Akata’s entry into the field of explainability. For half a year, she worked in Darrell’s research group at Berkeley. And then, as an assistant professor in Amsterdam, Akata later continued to collaborate with the group and published papers at major conferences.
In 2019, Akata became Professor for Explainable Machine Learning at the University of Tübingen’s Cluster of Excellence “Machine Learning for Science.” When she took up the position, she was only 33 years old and had just been awarded an ERC Starting Grant, the most prestigious European award for young scientists. “I had been advised not to apply for the grant yet,” she recounts, “but when people tell me you can’t do it, it gives me more motivation to do it.”
Akata brings a “can-do” spirit, confidence in her own projects, and the literal ability to transcend borders to her group. This comes as no surprise. Akata grew up and studied in the Turkish university town of Edirne. Then she went on to study in Aachen and Grenoble. About a dozen PhD students and postdocs from various countries, from Denmark to China, are part of her core team. The group is also predominantly international, because Akata is part of the European network ELLIS and supervises doctoral students together with researchers from other European universities or companies. In addition, there are several young researchers from other universities with whom the group collaborates.
From medical applications to self-driving cars
All group members are working on different aspects of explainability. In line with the mission of the Cluster of Excellence “Machine Learning for Science,” some are examining AI applications in other scientific fields and the role explainability plays here, for example, in medical imaging, or in the fields of computer vision and self-driving cars. Audio-visual learning is also part of the group’s repertoire. They work on explainability for AI systems that recognize patterns in audio and video.
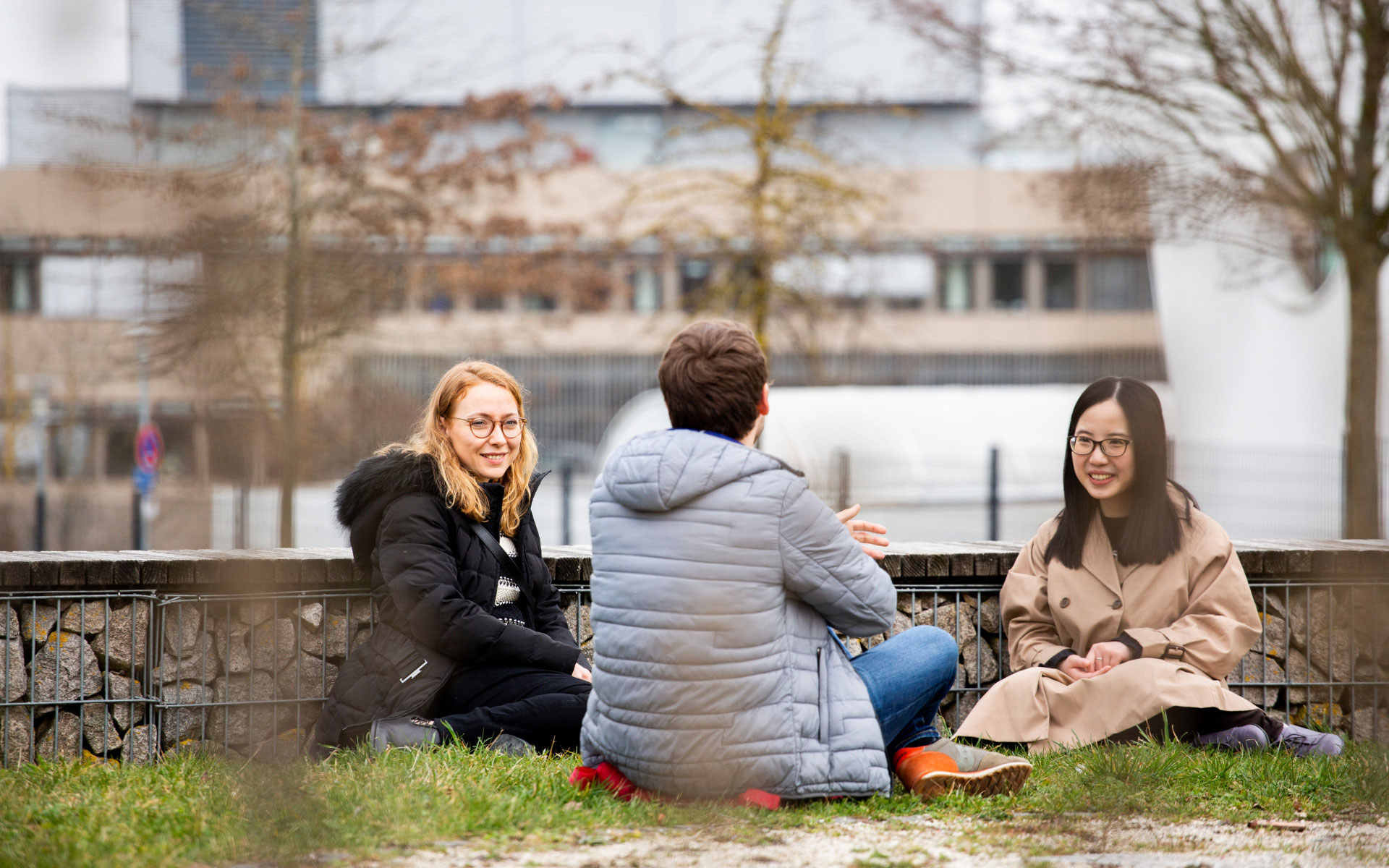
Encouraging her team to believe in their own goals: Zeynep Akata (left), here talking to her postdocs Massimiliano Mancini (center) and Yanbei Chen (right). © SOPHIA CARRARA/UNIVERSITY OF TÜBINGEN
This diversity reflects how strongly the field of explainability is now resonating in other scientific fields and industrial research. Could explainability become a key discipline that leads to greater transparency of AI systems in general? “I think calling a chair at the university ‘Explainable Machine Learning Chair’ is a bold move,” Akata says. “Explainability research forces researchers to think about the user’s perspective. Because we should try to understand why a certain model is working when it does – and why it is not working when it doesn’t.”
To find out more about the research of the Explainable Machine Learning group, please visit their website. For news, follow their twitter account.
Zeynep Akata was a member of our cluster and held the cluster W3-professorship for “Explainable Machine Learning” from 2019 to 2023. She is now professor for Computer Science at the Technical University of Munich and director of the Institute for Explainable Machine Learning at Helmholtz Munich.
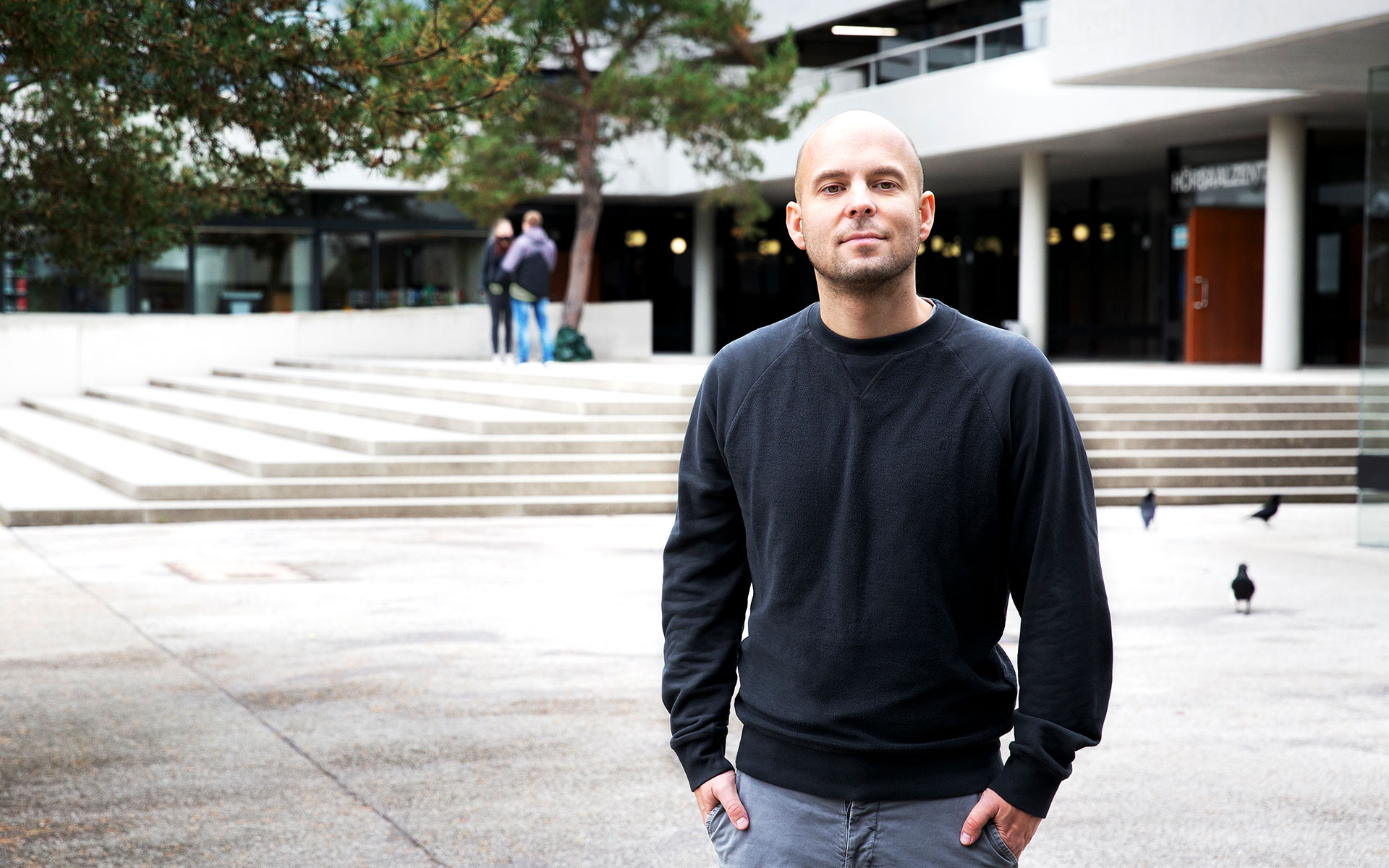
Where Algorithms and People Are Allies
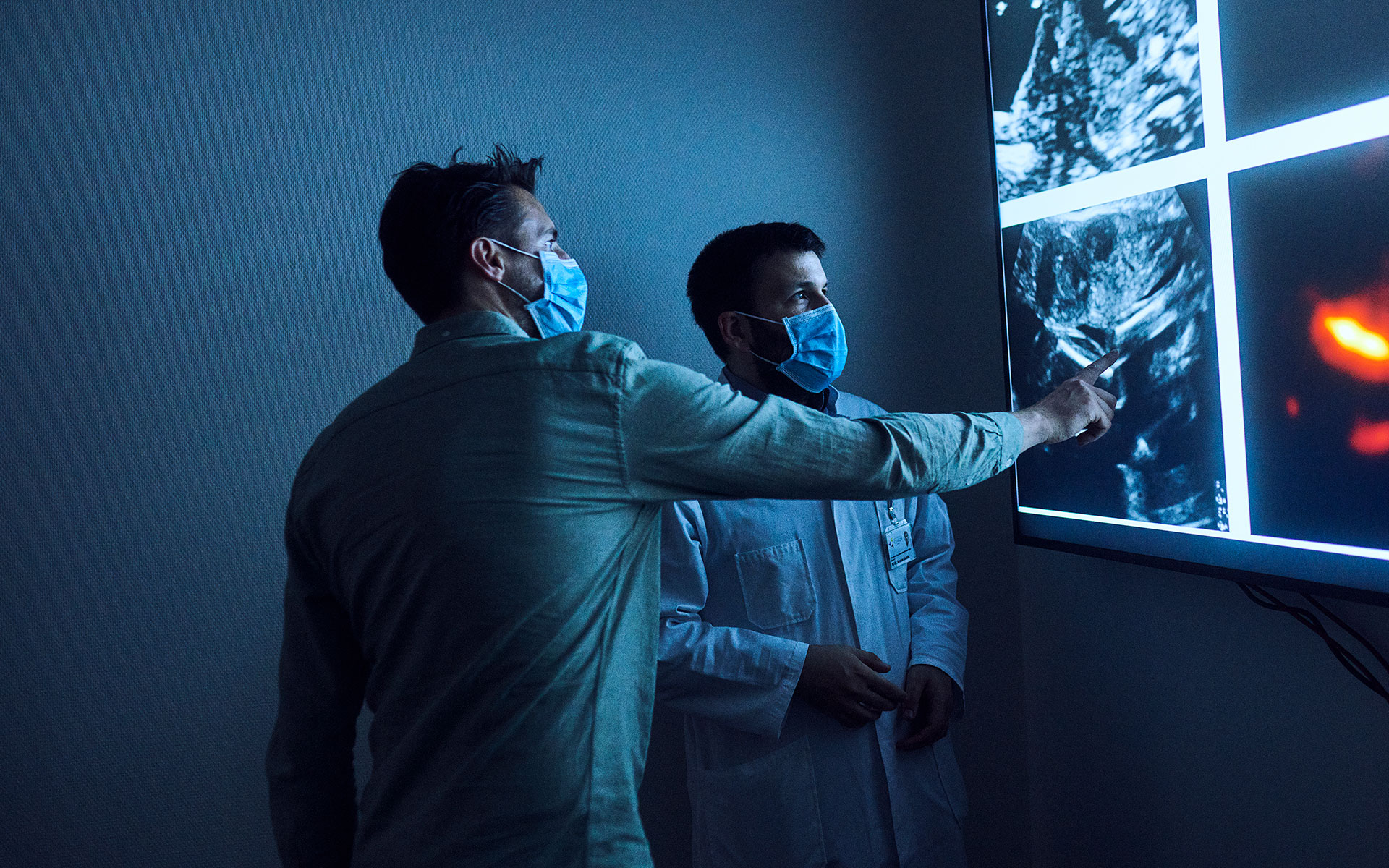
Comments