During the monsoon season, the Brahmaputra River in India turns into a sea. Rain as strong as a shower often pours down for days. Individual drops combine to form jets, and flooding often occurs. Bedartha Goswami grew up just a few hundred metres from the river, in the city of Guwahati in eastern India. He can still remember the monsoon’s torrents. In April of every year the rains spilled down – sometimes so heavily that school was cancelled. Then everyone would sit at home and watch the water splash on the leaves of the trees. But that was a long time ago. Goswami is now a bearded 37-year-old with a doctoral degree. The monsoon has also changed since then and has become more unpredictable. Sometimes it comes earlier, sometimes later. Sometimes the rains are heavier and destroy crops, sometimes there is not enough water. These are the effects of climate change. For many in India, these effects pose a huge problem because agriculture is the people’s livelihood. Therefore, one of the goals of Goswami and his research team at the “Machine Learning” Cluster of Excellence in Tübingen is to make the monsoon more predictable again – with the help of machine learning (ML).
Expanding the climate knowledge base
Climate change is also noticeable in Germany now, summers are hot and dry and extreme weather events are increasing. It is becoming clear that we need to act. Goswami decided to take the position at the Cluster of Excellence because he also wants to contribute to expanding the knowledge base on climate change. He wants to detect patterns in data and optimise models in order to make better predictions about the weather and the climate. That’s why he is going through huge climate data sets, looking for connections between rain, currents, and winds. A whiteboard with mathematical formulas hangs on the wall in his office, and in a cabinet, there are stacks of books with titles like Climate System Modelling, Machine Learning, and Dynamics of the Tropical Atmosphere and Oceans.
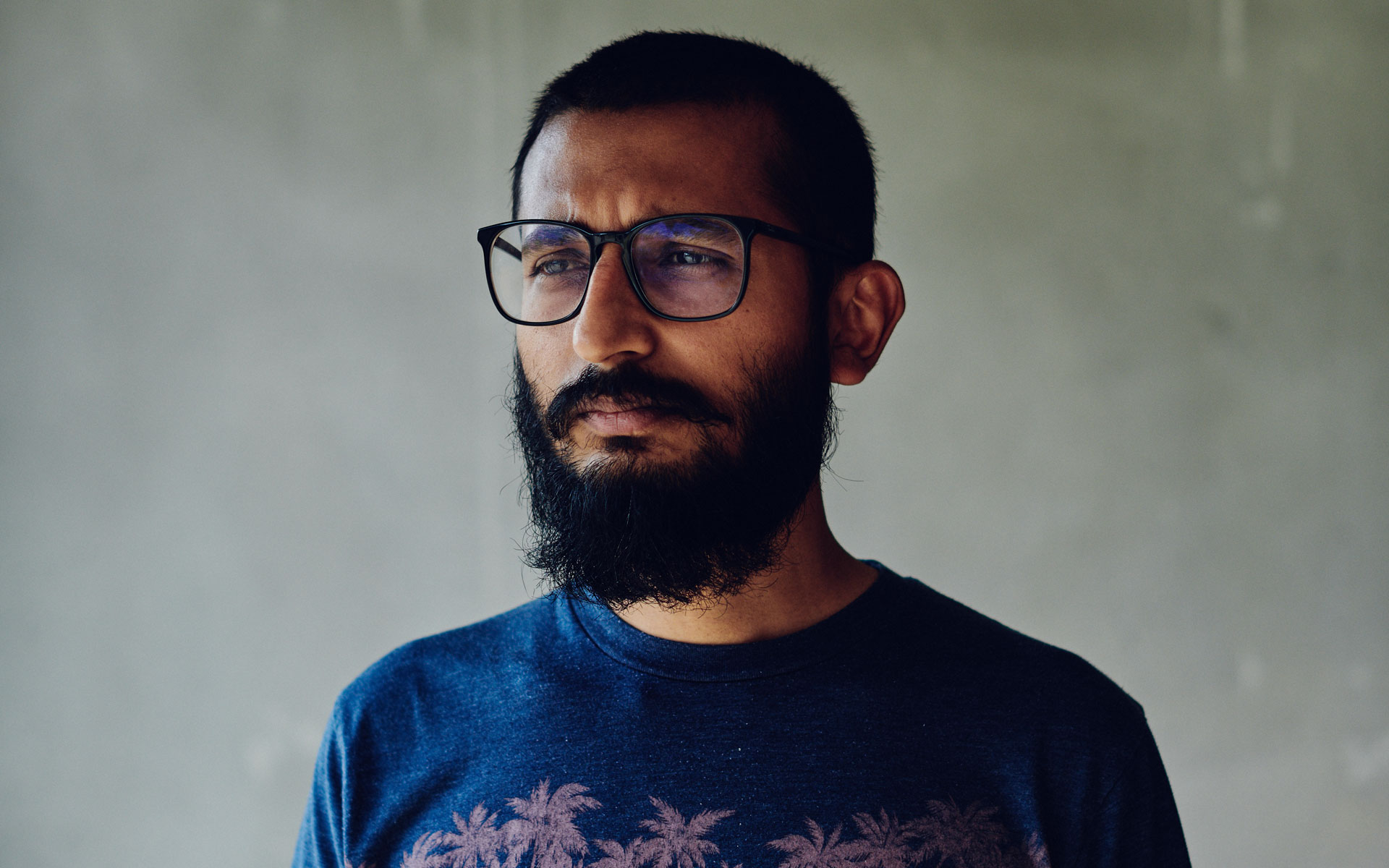
Since 2020 Bedartha Goswami has been head of the “Machine Learning in Climate Science” group at the University of Tübingen. © ELIA SCHMID / UNIVERSITY OF TÜBINGEN
But Goswami does not have a typical computer science background. In India he studied at the Indian Institute of Science Education and Research in Pune and did his doctoral work at the Potsdam Institute for Climate Research in Germany. There he learned how to analyse climate data, worked extensively with statistics, immersed himself in chaos theory, and dealt with complex systems. Still, many models and methods of ML research, especially in the field of deep learning, were initially new to him. Moreover, this branch of research continues to develop rapidly – so keeping up with it remains a challenge. Sitting in his office, Goswami says: “We have a lot to learn if we want to be at the forefront.” When he looks at other scientific papers that have attempted to build a bridge between machine learning and climate science, from time to time he sees that either the ML methods or the climate knowledge has been greatly simplified. They use outdated models or haven’t given enough thought to how climate science would benefit. But will he and his team of four PhD students be able to properly deal with both sides equally well?
Machine learning alone is not enough
Goswami leans back and says: “I always try to encourage my students to also engage with climate science in depth.” His motto is: “Quality over quantity.” If you ask the PhD students what they think of Goswami, his attention to detail comes up again and again. He deals with problems in detail. For him, acquiring knowledge is what counts. As a result, he wants to understand processes in detail, and he also wants to pass this knowledge along to his students. His goal is for them to become experts on certain aspects of the climate. That’s why he sends them to workshops, conferences, and summer schools. Moreover, he wants them to focus on the relevance of their publications rather than being the first to publish something. And he places priority on publishing in journals that are also important for meteorologists and climate scientists, not only for machine learning experts. In order to do this, he and his team also have to do the necessary reading so that, unlike many others, they can build a sufficiently solid bridge between ML and climate science. Sometimes it takes time before they’re ready to publish. Now, however, their first relevant studies have appeared.
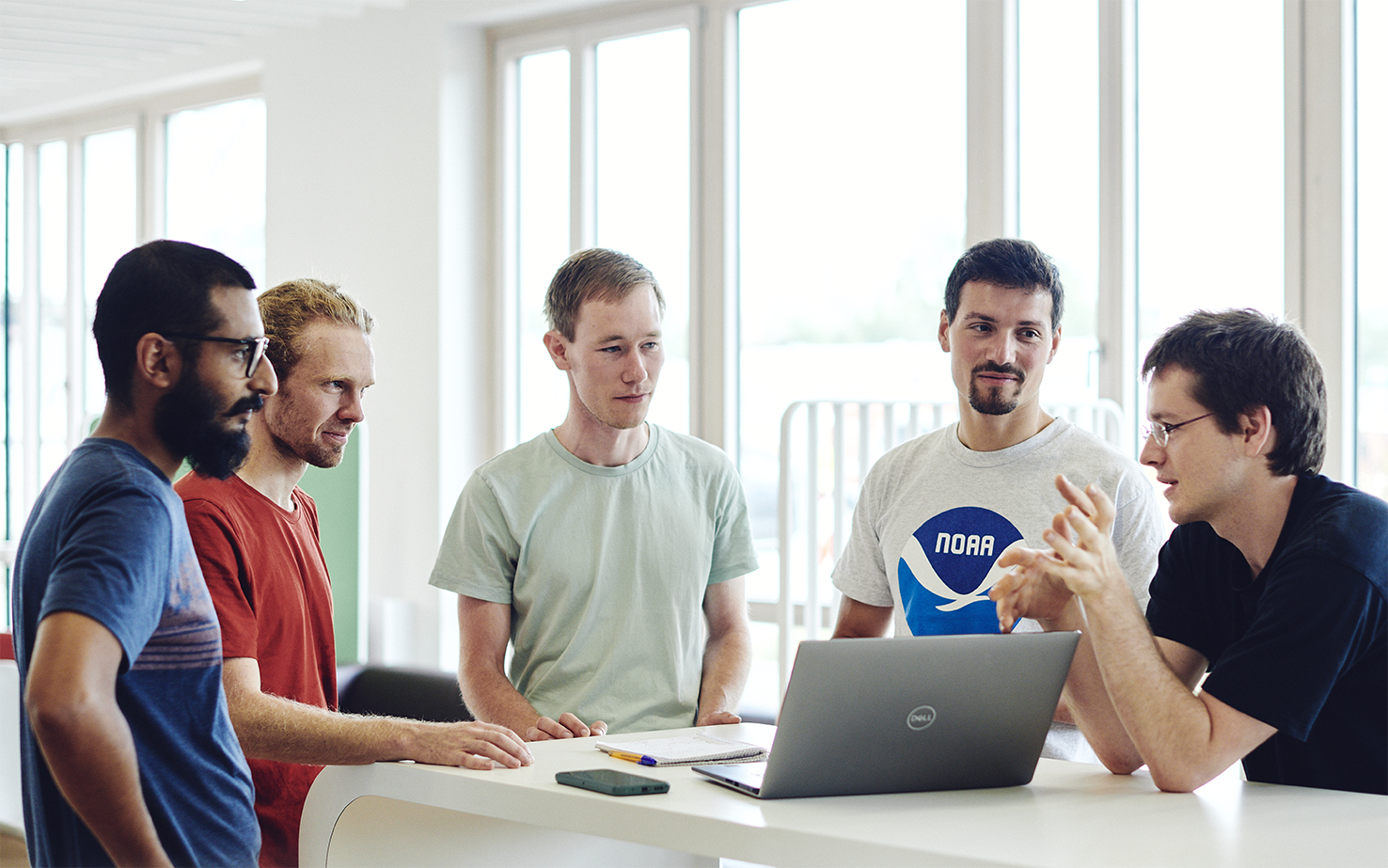
Bedartha Goswami talking to his doctoral students Felix Strnad, Moritz Haas, Jakob Schlör and Jannik Thümmel (from left to right). © ELIA SCHMID / UNIVERSITY OF TÜBINGEN
One example is the work of Jannik Thümmel, who develops predictive models using machine learning. He is working on a model that examines old weather data to learn how winds, rain, and storms are related, and then uses this as a basis to make predictions about future weather. In recent years, this approach has proven to be very successful. Before Thümmel accepted the position at the Cluster of Excellence, he had already done a good deal of work on deep learning. He studied cognitive science and computational neuroscience, and is the group’s in-house expert. Goswami says, “If anyone in our group has a problem with deep learning, they ask Jannik. He knows all the tips and tricks.” But that’s not enough to conduct relevant research. Thümmel also needs to become an expert in certain areas of climate science. That’s why he spent much of his first year at the Cluster of Excellence reading up on complex climate systems. The model that Thümmel is now developing is intended to help predict droughts or floods more precisely in the future. This would enable governments in the affected areas to take precautionary measures on a timely basis.
Every group member contributes a unique perspective
Along with Thümmel, Moritz Haas is also heavily involved in deep learning. He studied mathematics, but is now getting into the theory of machine learning. Unlike Thümmel, he belongs to the “Machine Learning Theory” research group headed by Ulrike von Luxburg; Goswami is his second supervisor. In his doctoral thesis, Haas is looking for weaknesses in machine learning methods in order to improve them, for example, through bias corrections and finding ways for ML models to avoid learning incorrect structures. The corrected methods then make possible more statistically reliable conclusions about complex structures in climate systems. Because Haas moves a lot within other research circles, he often comes up with an aspect or a piece of research that is new for a part of the group, which significantly advances their work. Goswami says, “Moritz is indispensable to the team” .
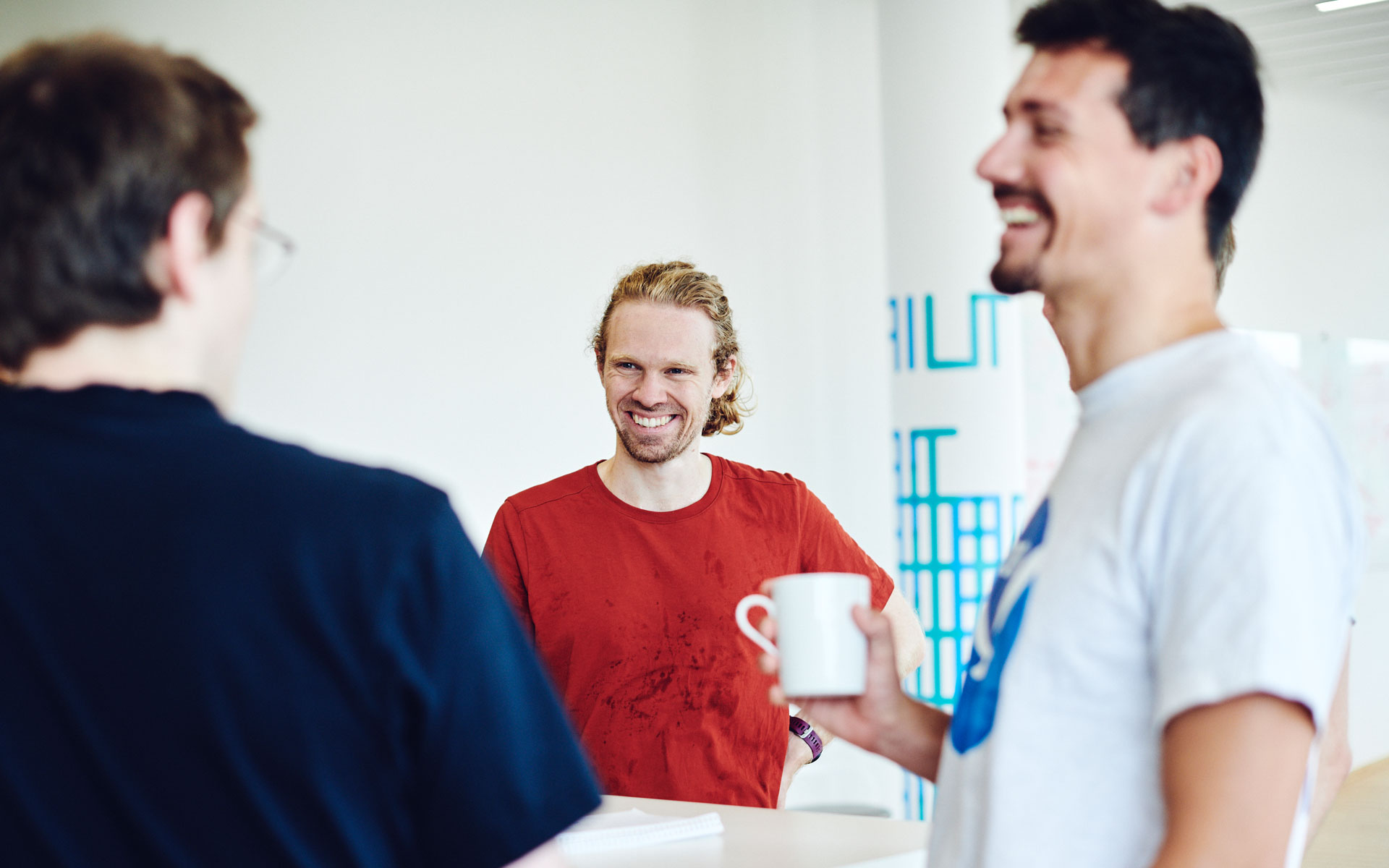
Each group member has a different scientific background – they learn from each other and support each other. © ELIA SCHMID / UNIVERSITY OF TÜBINGEN
And there is also Jakob Schlör, whose doctoral thesis sets out to better understand El Niño – the occurrence of unusual ocean currents in the Pacific. During an El Niño, the upper water layer of the tropical Pacific warms up to such an extent that it affects the global climate. The consequences include droughts in Australia, a weaker monsoon in India, and the collapse of entire food chains in the ocean. On average, the phenomenon occurs every two to seven years. But what factors occur before an El Niño year? And how are they changing as a result of climate change? As a physics student, Schlör showed a penchant for solving difficult mathematical problems. He wanted to combine this passion with work that was more meaningful . And in fact, El Niño can be seen as part of a mathematical problem. On one side of the equation are the unknown variables, and on the other is the occurrence of El Niño. In order to be able to solve the equation, Schlör has immersed himself in research areas that were completely new to him. During his stay as a visiting researcher at the American weather service NOAA, he became an expert in the stochastic modelling of climate systems. This knowledge is now helping him to improve forecast models for El Niño phenomena.
A New method for “early warning” forecasts
Last but not least there is Felix Strnad. He is seeking to develop a method to make “early warning” forecasts about when extreme precipitation events will occur in Asia. To that end, he is carrying out data analysis in the Indo-Pacific monsoon region. During his studies he already conducted research on the connection between complex systems and the climate. He studied physics in Göttingen and did his master’s thesis at the Potsdam Institute for Climate Research. Now, at the Cluster of Excellence, he is steadily making inroads into both worlds. He reads blog posts, watches tutorials, and searches for answers in online reference books. He sees himself as a mediator between the two worlds, a translator between machine learning and climate science. Using ML methods, he has discovered a new pattern in heavy rainfall in the Indo-Pacific region. He has discovered that the pathways of rainfall propagation fall into one of three categories: one that moves mainly northeast (in normal years); one that only migrates north (La-Nina); and a third that remains stationary (El Niño). Goswami is convinced that this discovery could amount to a significant breakthrough.
Building on this work, as well as on Schlör’s and Thümmel’s work, climate scientists could make the Indian monsoon a bit more predictable again. Back in his office, Goswami remarks, “With our work, we are making a small contribution to the expansion of knowledge about climate change.”
To find out more about the research of the Machine Learning in Climate Science group, please visit their website.
Text: Sarah Bioly
Translation into English: Fortuna Communication
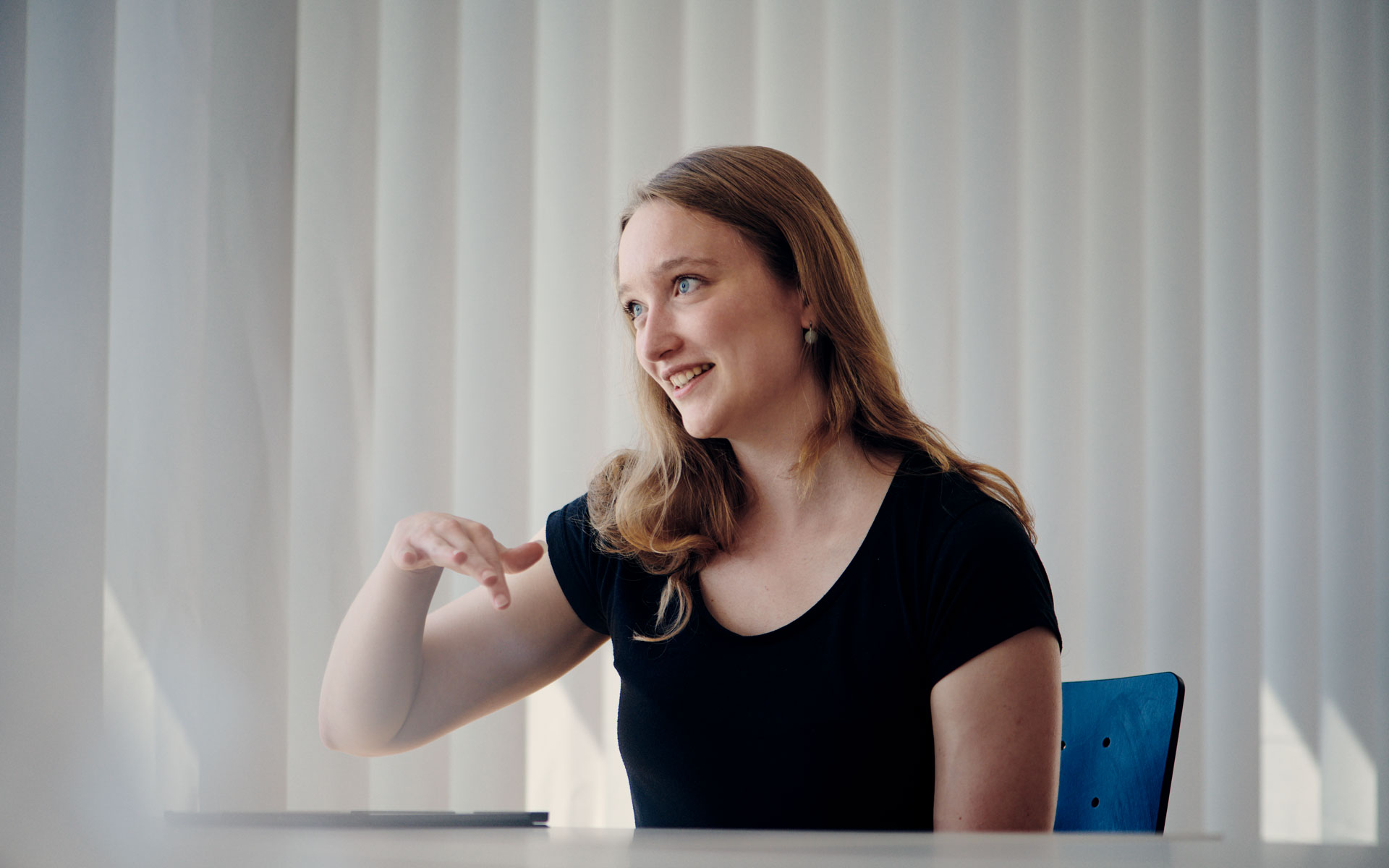
Predicting future energy supply
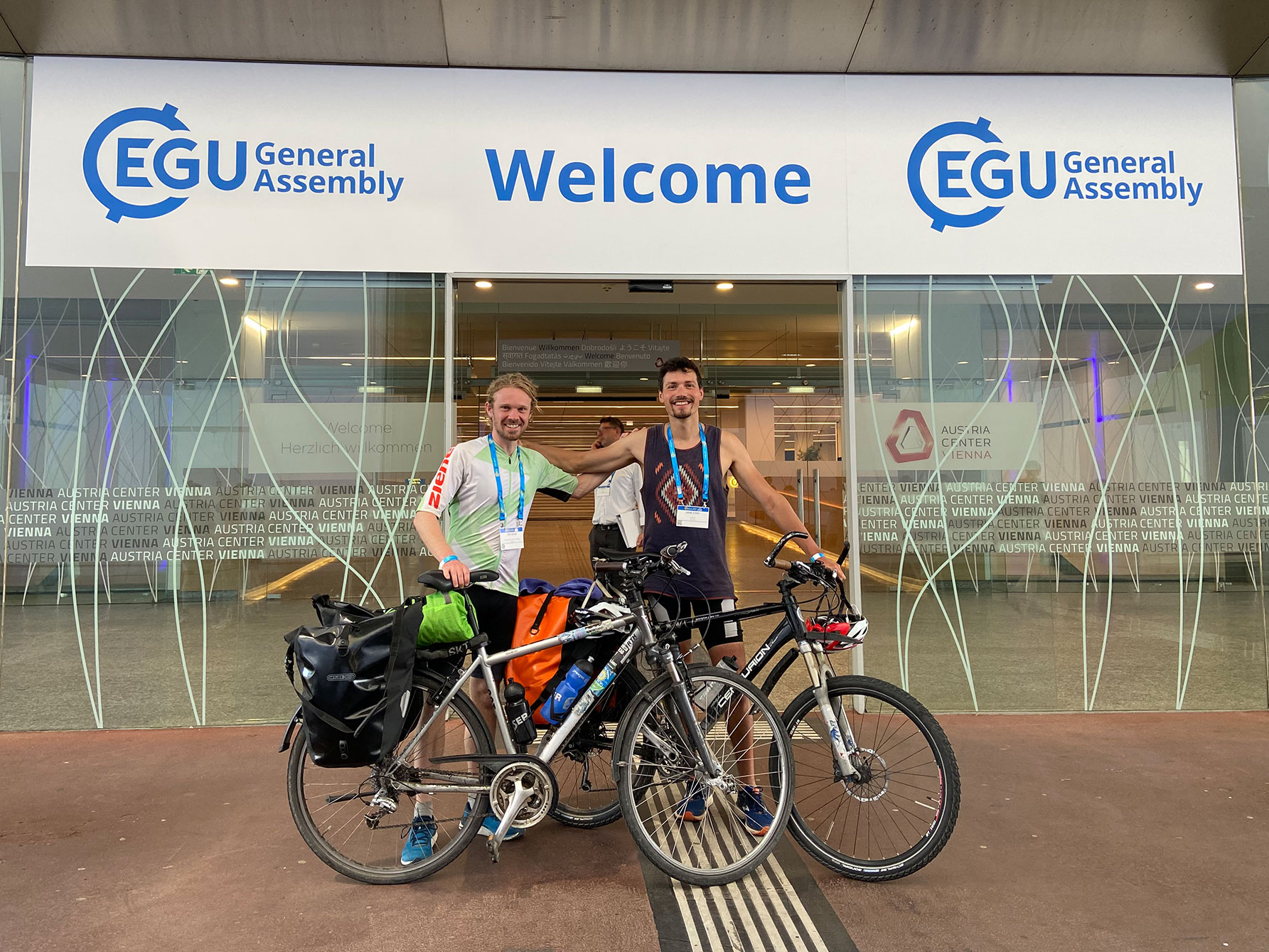
How to make business trips more climate friendly
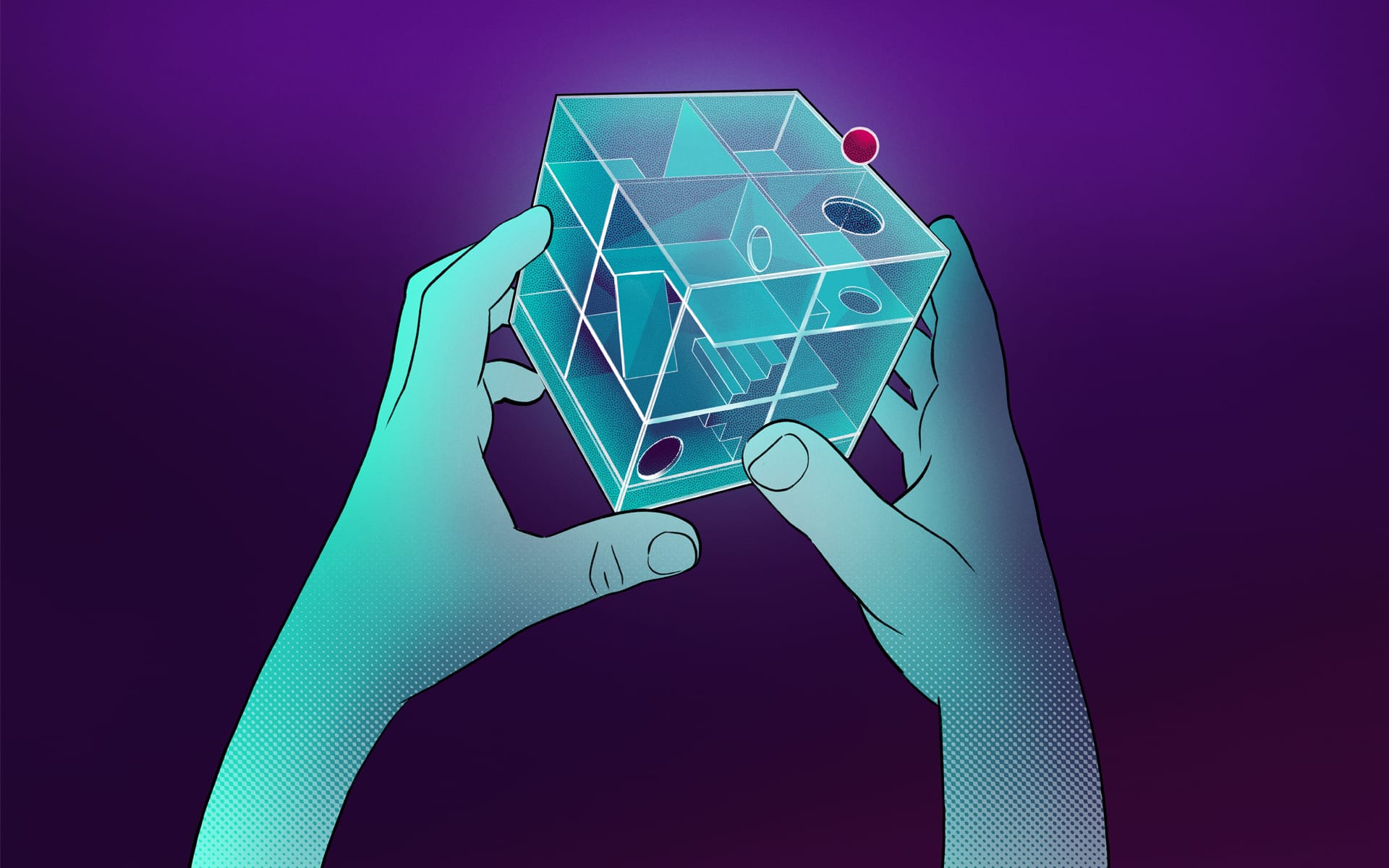
Comments