Why is it important to map soil?
Soils vary in space. They vary across the globe depending on climatic conditions and within landscapes depending on vegetation and landscape morphology. Soils even vary within individual agricultural fields in relation to the local geology and its hydrological, physical, and chemical characteristics. These variations challenge farmers as their daily business depends on their knowledge about each field and its local properties. For instance, too little or too much water can result in reduced plant growth and therefore less harvest. The same applies for other soil properties such as plant nutrients, e.g. nitrogen or potassium, if they differ from optimal conditions. Thus, it is beneficial for farmers to gather spatial knowledge about a field’s soil properties to exactly know where and to what extent fertilizer or irrigation must be applied to the respective field. This leads to optimal plant growth and reduced costs. It can also contribute to sustainable and environmentally friendly agriculture.
In our study published in the Vadose Zone Journal, we demonstrate how machine learning methods can be combined with cutting-edge techniques of geophysics and soil sciences. With our interdisciplinary team of soil scientists, geographers, geophysicists, and machine learning experts from the University of Tübingen and the Helmholtz Centre for Environmental Research in Leipzig we used geophysical sensors and machine learning to map soil properties in 3D across an agricultural field at the Elbe river in eastern Germany.
How can geophysics and machine learning make a difference?
Geophysical sensors provide insights in the spatial variation of the soil, for example by measuring the electrical conductivity, which is closely related to soil moisture and soil organic carbon content and can therefore be used to map the plants’ water and nutrient supply. Geophysical sensors allow collecting data in a cost- and time-efficient way, which can then be used for machine learning approaches to map other soil properties at high spatial resolution, such as soil moisture and soil organic carbon.
The electromagnetic induction sensors that we used in this study measure the electrical conductivity in three depth intervals at a time. We trained a machine learning model with the geophysical measurements describing the spatial and vertical variation of soil moisture and soil organic carbon based on ground truth data. With the trained model we predicted the soil properties to the whole feature space given by the geophysical measurements in high vertical resolution and with high accuracy – a 3D map of soil moisture and soil organic carbon. To achieve a comparably high resolution, elaborate and costly lab analysis would be necessary with conventional soil mapping.
For farmers, such 3D predictions are of great benefit: They can plan the irrigation and fertilization of their fields much more efficiently.
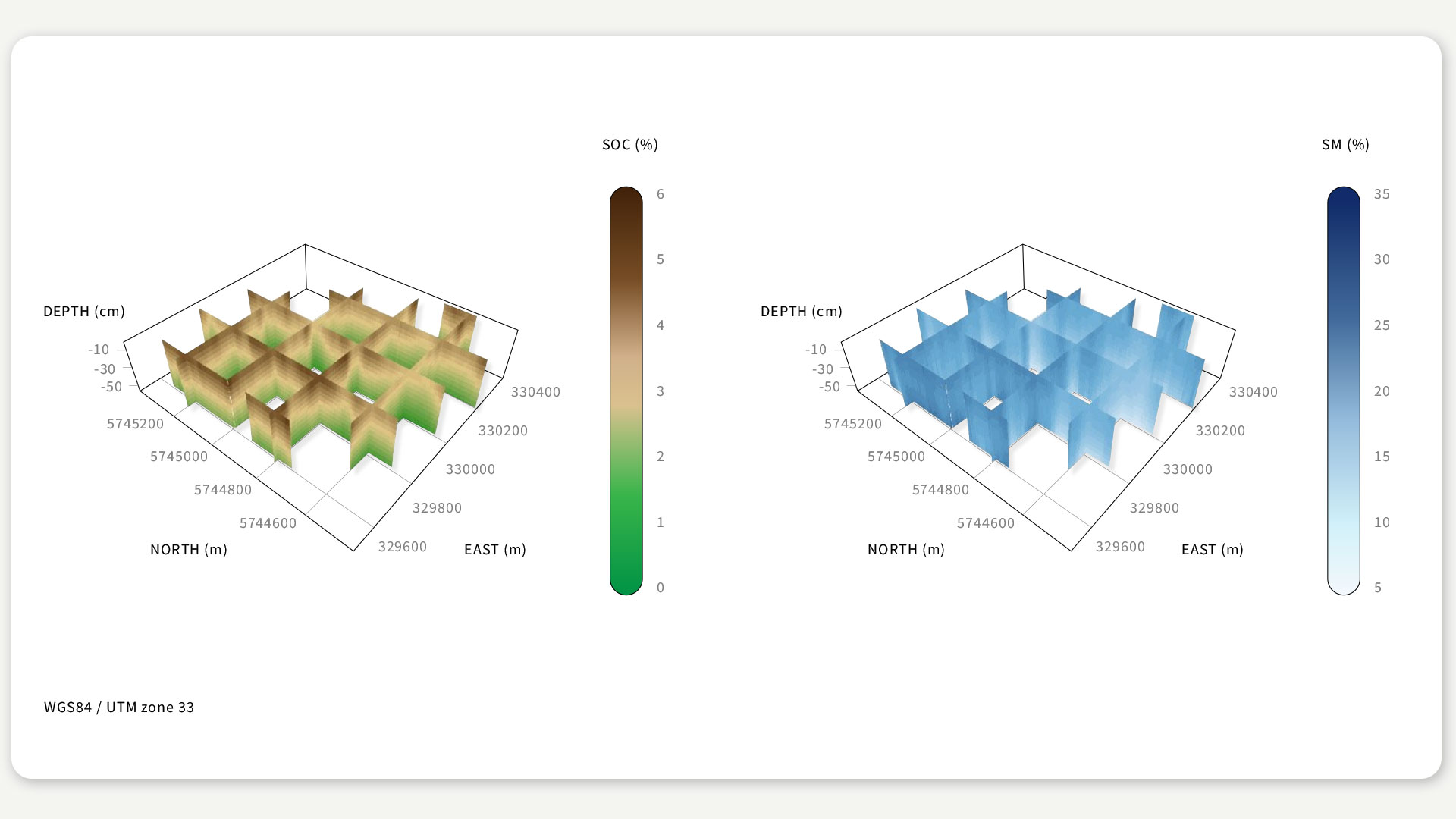
Figure: 3D predictions of soil organic carbon (SOC) and soil moisture (SM). © According to Rentschler et al, 2020.
What next?
A next possible step is the modelling of soil property variation over time since soil moisture depends on the weather conditions and the soil organic carbon on land use during the growing season. These investigations require a stable repeatability of the geophysical measurements and careful calibration of the time series. This spatial-temporal mapping of soil properties with machine learning techniques would be novel for soil sciences.
Text: Tobias Rentschler, Karsten Schmidt, Thomas Scholten, Sandra Teuber, Ulrike Werban
Cover illustration: photo: Department of Monitoring and Exploration Technologies, Helmholtz Centre for Environmental Research – UFZ; graphical editing: Franz-Georg Stämmele
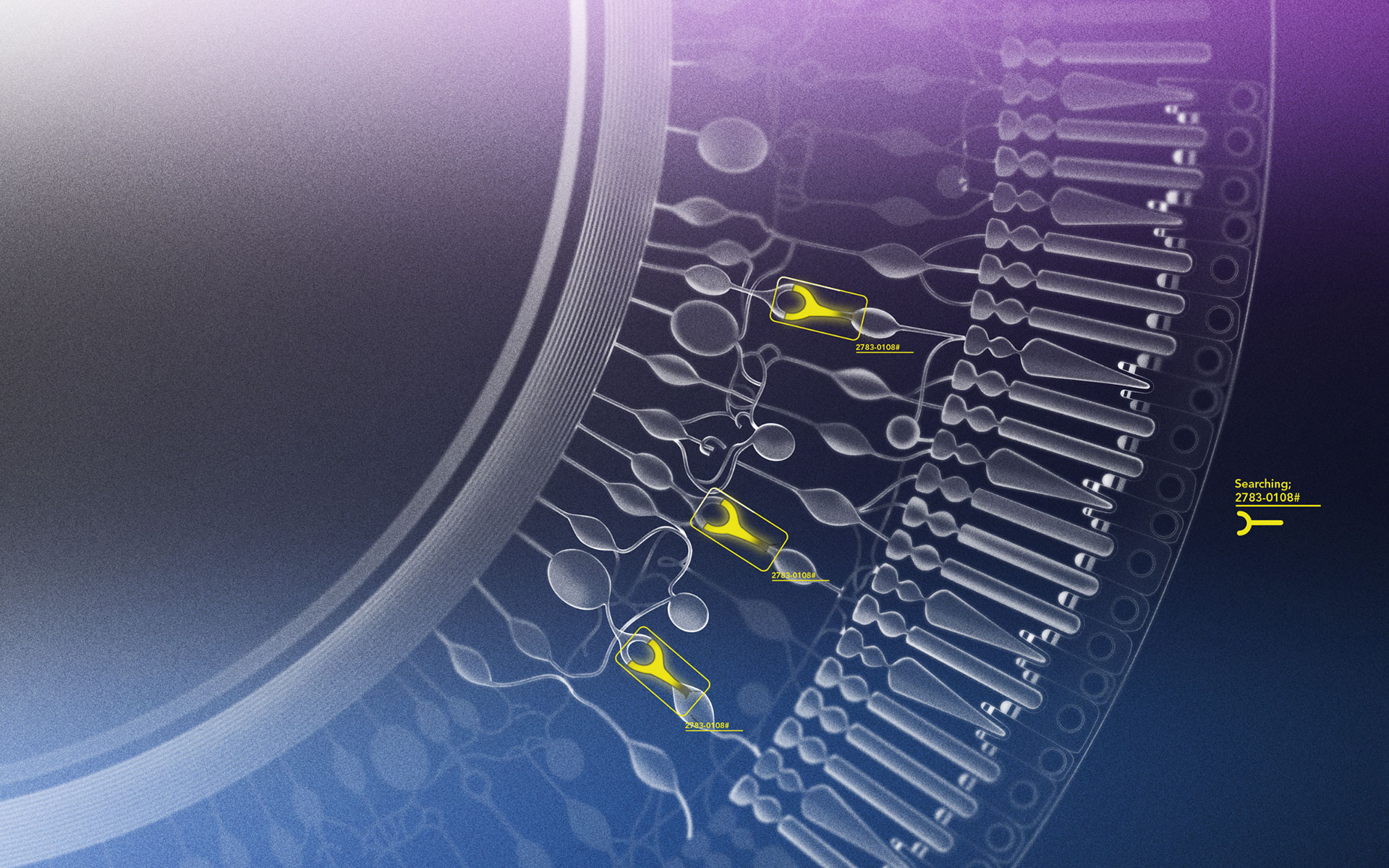
Identifying Models in
Neuroscience with Machine Learning
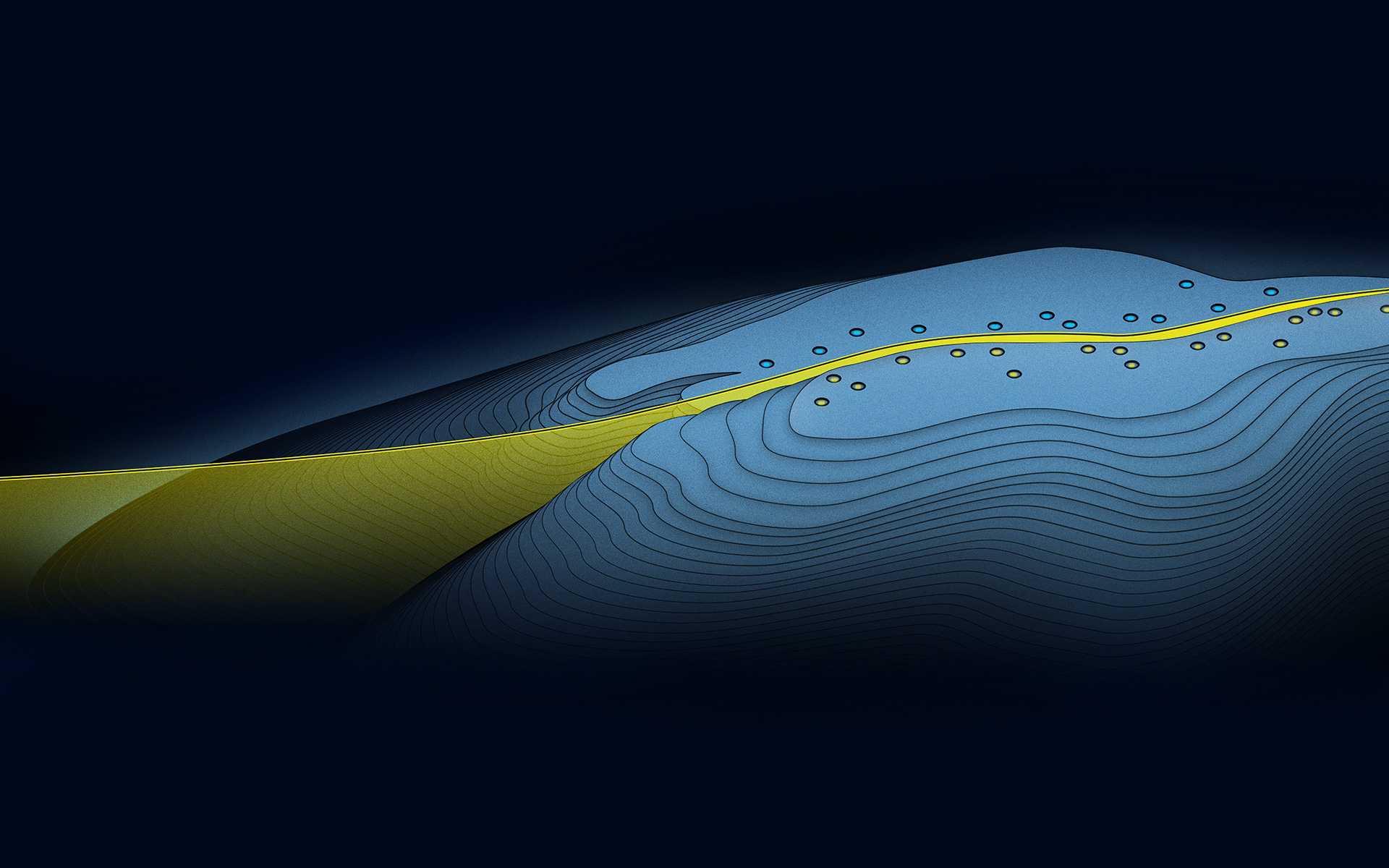
Comments